Method for detecting and classifying defects of non-woven fabrics
A technology of defect detection and classification method, applied in the field of image recognition, can solve the problems of large number of training samples and difficult implementation, and achieve the effect of high classification accuracy and reduced running time.
- Summary
- Abstract
- Description
- Claims
- Application Information
AI Technical Summary
Problems solved by technology
Method used
Image
Examples
Embodiment Construction
[0017] The present invention will be described in further detail below with reference to the accompanying drawings and specific embodiments.
[0018] In order to quickly locate the defect area in the non-woven defect image and accurately classify the defect type, the present invention proposes an automatic detection and classification method for non-woven defects, so as to realize the automation of non-woven quality inspection.
[0019] In step 1, a grayscale image of the non-woven fabric is obtained from the non-woven fabric inspection production line, and the image can be obtained by an ordinary industrial camera. The defect pictures are selected, and a total of 4 kinds of defects such as holes, oil stains, foreign objects and scratches are collected, such as figure 2 , each type of defect can include 50 pictures, all pictures can be 8-bit grayscale images, and the size can be 1280×960. Each class can use 80% of the randomly selected images as training samples, and all ima...
PUM
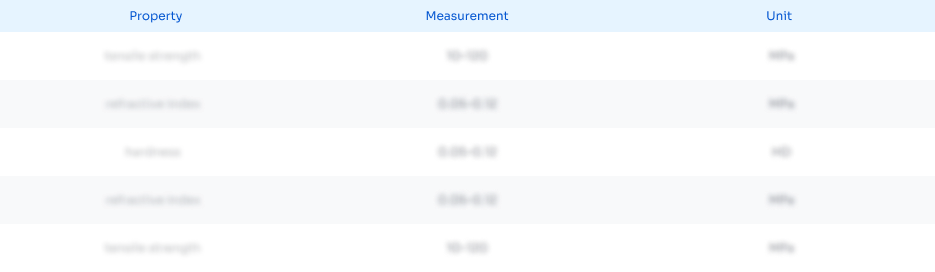
Abstract
Description
Claims
Application Information
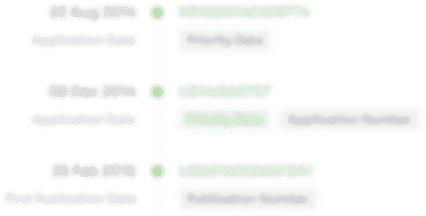
- R&D Engineer
- R&D Manager
- IP Professional
- Industry Leading Data Capabilities
- Powerful AI technology
- Patent DNA Extraction
Browse by: Latest US Patents, China's latest patents, Technical Efficacy Thesaurus, Application Domain, Technology Topic, Popular Technical Reports.
© 2024 PatSnap. All rights reserved.Legal|Privacy policy|Modern Slavery Act Transparency Statement|Sitemap|About US| Contact US: help@patsnap.com