Deep learning-based unusual speech distinguishing method
A deep learning, abnormal technology, applied in speech recognition, speech analysis, instruments, etc., can solve the problems of speaker information interference and recognition performance degradation
- Summary
- Abstract
- Description
- Claims
- Application Information
AI Technical Summary
Problems solved by technology
Method used
Image
Examples
Embodiment 1
[0104] A method for distinguishing abnormal speech based on deep learning, comprising the following steps:
[0105] Step 1: Obtain the input voice, and perform preprocessing such as resampling, pre-emphasis, frame division and windowing on the input voice to obtain the pre-processed voice;
[0106] Resampling is specifically: the input voice has different sampling frequencies and encoding methods. In order to facilitate data processing and analysis, the original input voice signal is resampled, and the sampling frequency and encoding method are unified; the sampling frequency is 22.05kHz, and the encoding method is wav Format.
[0107] The pre-emphasis is specifically: the power spectrum of the audio signal decreases with the increase of the frequency, and most of the energy is concentrated in the low-frequency range. In order to improve the high-frequency part of the original audio signal, the original input audio signal is pre-emphasized. order FIR high-pass filter, its tra...
PUM
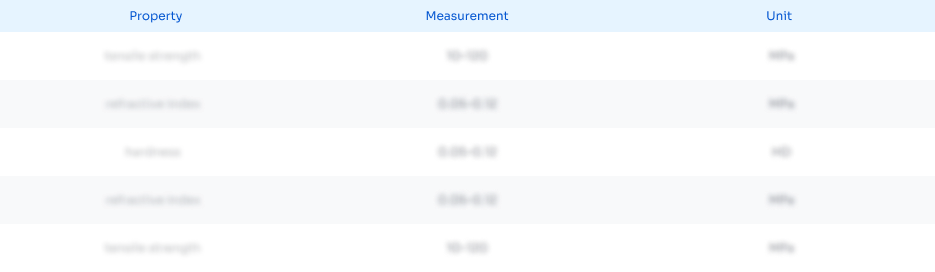
Abstract
Description
Claims
Application Information
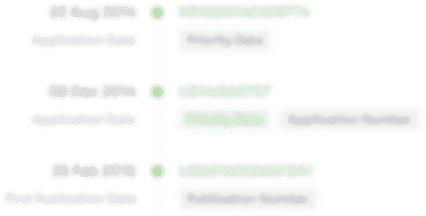
- R&D
- Intellectual Property
- Life Sciences
- Materials
- Tech Scout
- Unparalleled Data Quality
- Higher Quality Content
- 60% Fewer Hallucinations
Browse by: Latest US Patents, China's latest patents, Technical Efficacy Thesaurus, Application Domain, Technology Topic, Popular Technical Reports.
© 2025 PatSnap. All rights reserved.Legal|Privacy policy|Modern Slavery Act Transparency Statement|Sitemap|About US| Contact US: help@patsnap.com