Time sequence anomaly point detection method and device
A time series and anomaly detection technology, applied in the field of data processing, can solve problems such as large prediction deviations, achieve the effects of improving accuracy, reducing prediction deviations, and reducing false alarm rates
- Summary
- Abstract
- Description
- Claims
- Application Information
AI Technical Summary
Problems solved by technology
Method used
Image
Examples
Embodiment 1
[0046] Such as figure 1 As shown, the time series outlier detection method provided by the embodiment of the present invention may include the following steps:
[0047] Step S101: using the training set T to train the regression model of the time series;
[0048] Step S102: Predict the current time series value according to the regression model obtained from training and the input time series one period before the current time, and perform anomaly detection on the observed current time series value according to the predicted current time series value. Preferably, in step S102, it is judged whether the difference between the predicted current time series value and the observed current time series value is greater than a preset threshold, if yes, the observed current time series value is considered to be abnormal; otherwise, the observed current time series value is considered to be abnormal. The current time sequence value of is normal.
[0049]Step S103: According to the res...
Embodiment 2
[0054] On the basis of the time series outlier detection method provided in Embodiment 1, the discrimination threshold of the outlier is optimized, wherein:
[0055] Step S101 also includes: calculating the standard deviation σ of the training set;
[0056] The preset threshold used in step S102 is determined by the following formula:
[0057] D=3σ;
[0058] Where D is the preset threshold, and σ is the standard deviation of the training set.
[0059] In the anomaly detection stage, the choice of threshold has an important impact on the determination of outliers. If the threshold is too large, the false positive rate will increase; if the threshold is too small, many false positives will appear. Only by selecting the threshold reasonably can the outliers be judged correctly, making subsequent replacement strategies more successful. In threshold selection, this method adopts 3σ criterion. When the sample is large enough, it can be approximately considered as a normal distr...
Embodiment 3
[0062] The present invention provides the time series abnormal point detection method of the third embodiment in combination with the foregoing embodiments one and two, and the specific implementation process is as follows:
[0063] Step S201: the process starts;
[0064] Step S202: Establish a regression model Regressor, and use the training set T to train the regression model;
[0065] Step S203: Calculate the standard deviation σ of the training set;
[0066] Step S204: Let the abnormal point set P be an empty set;
[0067] Step S205: set the time sequence number index=k+1; k is the input length of the regression model.
[0068] Step S206: Determine whether the time sequence number index is less than or equal to the total length of the time series S, if yes, go to step S207, otherwise go to step S212;
[0069] Step S207: The period of time series [S in the time series T index-k-1 ,...,S index-1 ] Input the regression model Regressor, and predict the sequence value pred...
PUM
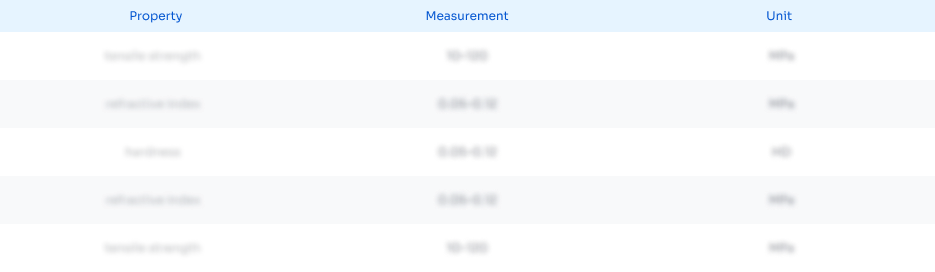
Abstract
Description
Claims
Application Information
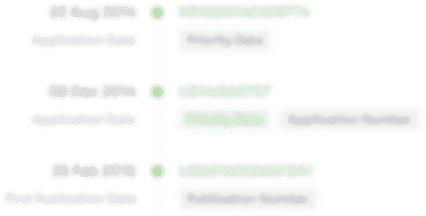
- R&D
- Intellectual Property
- Life Sciences
- Materials
- Tech Scout
- Unparalleled Data Quality
- Higher Quality Content
- 60% Fewer Hallucinations
Browse by: Latest US Patents, China's latest patents, Technical Efficacy Thesaurus, Application Domain, Technology Topic, Popular Technical Reports.
© 2025 PatSnap. All rights reserved.Legal|Privacy policy|Modern Slavery Act Transparency Statement|Sitemap|About US| Contact US: help@patsnap.com