Method for intelligently diagnosing rotating machine fault feature based on deep CNN model
A deep convolution and network structure technology, applied in neural learning methods, biological neural network models, neural architectures, etc., can solve the problems of expressing useful information of vibration signals, but cannot reflect time-varying characteristic information, etc., and achieve excellent performance and strong information. Capture and Characterization Capability, Avoid Spectrum Leakage Effects
- Summary
- Abstract
- Description
- Claims
- Application Information
AI Technical Summary
Problems solved by technology
Method used
Image
Examples
Embodiment Construction
[0045]A method for intelligently diagnosing fault characteristics of rotating machinery based on a deep convolutional neural network structure of the present invention, the steps of which include: (1) collecting vibration signal test data for rotating machinery faults, reasonably segmenting the data and performing detrending item preprocessing; (2) Perform short-term Fourier time-frequency transformation analysis on the collected signal data to obtain the time-frequency characteristics of each vibration signal, and display it using a pseudo-color map; (3) use interpolation to reduce the image resolution and superimpose each image, Form training samples and test samples as the input of convolutional neural network; (4) construct deep convolutional neural network model, including input layer, two convolutional layers, two pooling layers, fully connected layer and classification layer and output (5) Import the training samples into the deep convolutional neural network model for t...
PUM
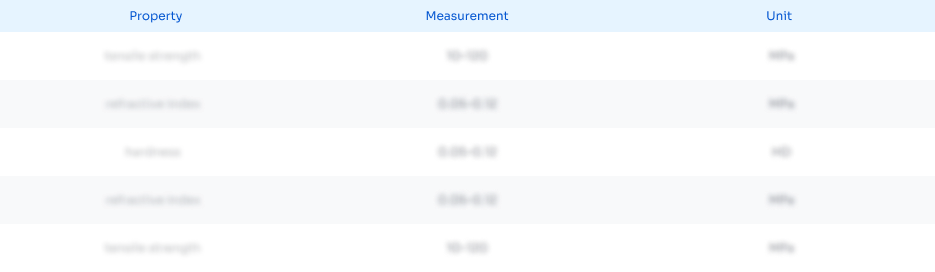
Abstract
Description
Claims
Application Information
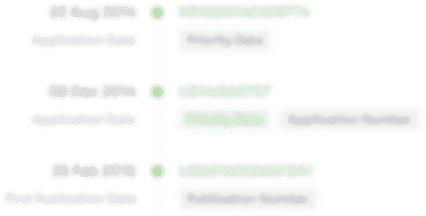
- R&D
- Intellectual Property
- Life Sciences
- Materials
- Tech Scout
- Unparalleled Data Quality
- Higher Quality Content
- 60% Fewer Hallucinations
Browse by: Latest US Patents, China's latest patents, Technical Efficacy Thesaurus, Application Domain, Technology Topic, Popular Technical Reports.
© 2025 PatSnap. All rights reserved.Legal|Privacy policy|Modern Slavery Act Transparency Statement|Sitemap|About US| Contact US: help@patsnap.com