Polysemous-word word vector disambiguation method
A word vector and polysemy technology, applied in the intersection of text mining and machine learning, can solve the problems of sparse corpus features, low accuracy, and high corpus dependence.
- Summary
- Abstract
- Description
- Claims
- Application Information
AI Technical Summary
Problems solved by technology
Method used
Image
Examples
Embodiment 1
[0020] Example 1: Dataset composition
[0021] The data set of this experiment is divided into two parts, one is e-commerce comment data and Simplified Chinese Wikipedia corpus, and the other is Sogou news text classification corpus, the former is mainly used for qualitative evaluation, and the latter is used for quantitative evaluation evaluate.
[0022] First, in order to conduct a better qualitative evaluation of polysemous words, here is the combination of e-commerce review data and Simplified Chinese Wikipedia corpus, because there are relatively few polysemous words in text corpora in a single field, and it is difficult to evaluate polysemous words. Qualitative description of semantic phenomena, and Wikipedia has a wide range of fields, which is suitable for mining polysemy. The e-commerce review data has a total of 4,904,600 comments. After processing the Wikipedia corpus, a total of 361,668 entries and 1,576,564 lines were obtained after extracting the text content, c...
Embodiment 2
[0028] Embodiment 2: Polysemous word vector training
[0029] figure 1 As shown in the polysemous word vector algorithm, the word vector and topic vector are calculated separately, combined with the result word: (topic number) after BTM topic model training, the word vector and subject vector are calculated by the formula
[0030]
[0031] to connect, Represents the connection of word vector w and topic vector z, w z The length of is the sum of the lengths of word vector w and topic vector z. Here the length of the word vector and the length of the subject vector need not be the same.
[0032] BTM topic annotation. The parameter inference of the BTM topic model uses the Gibbs sampling process to obtain the hidden topic corresponding to each word in each document in the text set. At the same time, since the training effect of Skip-gram on a larger corpus is better than that of the CBOW model, this chapter uses Skip-gram training model here. The topic sampling formula ...
Embodiment 3
[0058] Embodiment 3: experimental verification process
[0059] (1) Quantitative verification
[0060] 1) Word vector similarity
[0061] Table 4 word2vec word vector similarity results
[0062]
[0063] Table 5 Polysemous word vector similarity results
[0064]
[0065] First, the e-commerce reviews and Wikipedia corpus are preprocessed, including removing stop words and word segmentation. In order to filter the network words in the corpus, the list of stop words is expanded here to reduce the noise of the corpus. At the same time, due to some frequency Lower words contain less information and affect the effect of word vectors, so words with a frequency lower than 5 are deleted. The word vector similarity mainly compares the word vector similarity between the polysemous word vector algorithm and the word2vec algorithm. The results are shown in Table 4 and Table 5.
[0066] Through the analysis of Table 4 and Table 5, the word2vec algorithm has only a single unique ...
PUM
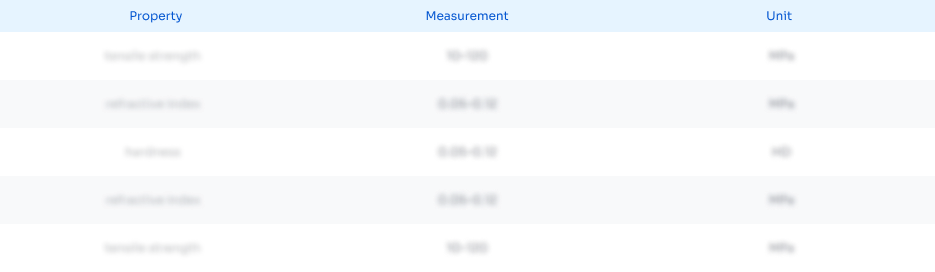
Abstract
Description
Claims
Application Information
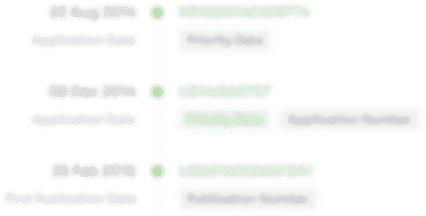
- R&D
- Intellectual Property
- Life Sciences
- Materials
- Tech Scout
- Unparalleled Data Quality
- Higher Quality Content
- 60% Fewer Hallucinations
Browse by: Latest US Patents, China's latest patents, Technical Efficacy Thesaurus, Application Domain, Technology Topic, Popular Technical Reports.
© 2025 PatSnap. All rights reserved.Legal|Privacy policy|Modern Slavery Act Transparency Statement|Sitemap|About US| Contact US: help@patsnap.com