Acquisition method and system of sample picture data set
A technology of data collection and sample pictures, applied in the field of data processing, can solve the problems of high subjectivity of screening results, error-prone screening results, wrong classification results, etc., to reduce labor and subjectivity, speed up training, and improve model accuracy Effect
- Summary
- Abstract
- Description
- Claims
- Application Information
AI Technical Summary
Problems solved by technology
Method used
Image
Examples
no. 1 example
[0056] The first embodiment of the present invention, such as figure 1 Shown:
[0057] A method for obtaining a sample picture data set, comprising:
[0058] The cleaning process of the image data to be cleaned specifically includes:
[0059] Obtain a positive sample picture set and a negative sample picture set; the feature information of the picture data in the positive sample picture set is the same as the feature information of the target picture; the feature information of the picture data in the negative sample picture set is different from the feature information of the target picture same;
[0060] Specifically, the feature information includes, but is not limited to, picture features such as picture content and picture category. The pictures crawled from the Internet can be manually screened with a preset number of picture data that is the same as the feature information of the target picture as a positive sample picture set, and manually screened with a preset num...
no. 3 example
[0074] The third embodiment of the present invention. This embodiment is a preferred embodiment of the first embodiment. Compared with the first embodiment above, it is further optimized. According to the positive sample picture set and the negative sample picture set, training is obtained A neural network sorter consists of steps:
[0075] Delete the last fully connected layer of the pre-trained neural network model;
[0076] Add a fully connected layer and an activation layer in turn after deleting the last fully connected layer;
[0077] The neural network sorter is obtained by training a newly added fully connected layer and an activation layer according to the positive sample picture set and the negative sample picture set.
[0078] Specifically, the operation of the transfer learning training sorter is actually to delete the last fully connected layer of the pre-trained neural network model such as (mobilenetv1), and then add a fully connected layer and an activation l...
no. 4 example
[0079] The fourth embodiment of the present invention. This embodiment is a preferred embodiment of the first embodiment. Compared with the first embodiment above, it is further optimized. According to the neural network sorter, the picture data to be cleaned is classified to obtain several Confidence set consists of steps:
[0080] Input all image data to be cleaned into the neural network sorter to obtain the confidence level of each image data to be cleaned;
[0081] Classify the image data to be cleaned into corresponding confidence sets according to the confidence of the image data to be cleaned and the preset confidence interval division range.
[0082] Specifically, after training the neural network sorter, all the image data to be cleaned are input into the neural network sorter, and the neural network sorter is used to predict each image data to be cleaned to obtain the corresponding confidence. Confidence represents the probability that the image data to be cleaned ...
PUM
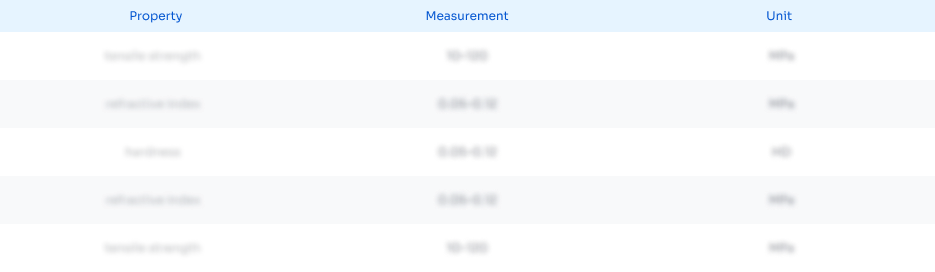
Abstract
Description
Claims
Application Information
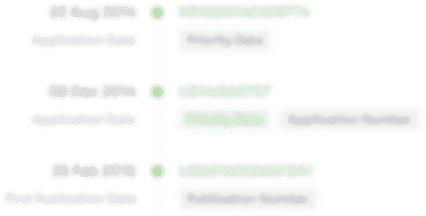
- R&D
- Intellectual Property
- Life Sciences
- Materials
- Tech Scout
- Unparalleled Data Quality
- Higher Quality Content
- 60% Fewer Hallucinations
Browse by: Latest US Patents, China's latest patents, Technical Efficacy Thesaurus, Application Domain, Technology Topic, Popular Technical Reports.
© 2025 PatSnap. All rights reserved.Legal|Privacy policy|Modern Slavery Act Transparency Statement|Sitemap|About US| Contact US: help@patsnap.com