A partial binary convolution method suitable for embedded devices
An embedded device and convolution method technology, applied in the direction of neural architecture, biological neural network model, etc., can solve the problems of decreased accuracy, unconsidered importance, etc., and achieve the effect of reducing calculations
- Summary
- Abstract
- Description
- Claims
- Application Information
AI Technical Summary
Problems solved by technology
Method used
Image
Examples
Embodiment Construction
[0022] Below in conjunction with accompanying drawing and embodiment the present invention will be further described:
[0023] The information processing process of the present invention is as figure 1 as shown, figure 1 Contains 4 subgraphs: convolution kernel grouping, convolution kernel rearrangement, channel rearrangement and model fine-tuning.
[0024] 1. The convolution kernel grouping subgraph is step 1 of the present invention, that is, for each convolution layer of a given depth convolutional neural network, the importance of each convolution kernel is calculated according to the convolution kernel importance calculation formula; And sort the convolution kernels of each layer according to the importance, set the convolution kernel importance threshold, and divide the convolution kernels larger than the threshold and smaller than the threshold into two groups. N in the figure c is the number of channels of the input feature map, h i / w i is the height and width of...
PUM
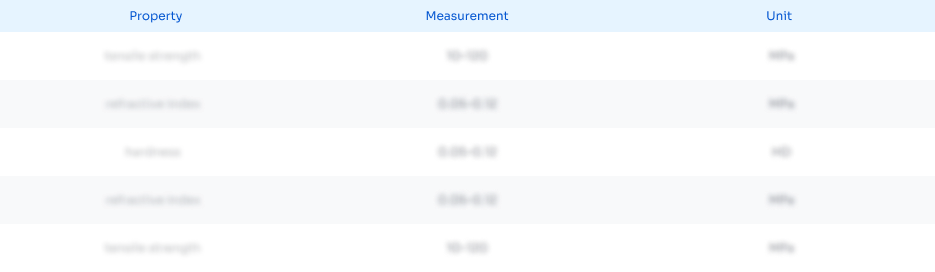
Abstract
Description
Claims
Application Information
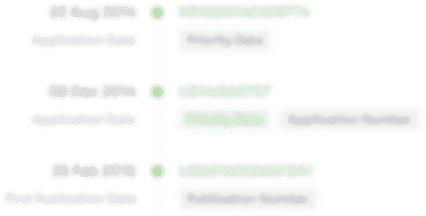
- R&D
- Intellectual Property
- Life Sciences
- Materials
- Tech Scout
- Unparalleled Data Quality
- Higher Quality Content
- 60% Fewer Hallucinations
Browse by: Latest US Patents, China's latest patents, Technical Efficacy Thesaurus, Application Domain, Technology Topic, Popular Technical Reports.
© 2025 PatSnap. All rights reserved.Legal|Privacy policy|Modern Slavery Act Transparency Statement|Sitemap|About US| Contact US: help@patsnap.com