An active learning method of hyperspectral image based on graph signal sampling
A hyperspectral image and signal sampling technology, applied in the fields of instruments, character and pattern recognition, computer parts, etc., can solve the problem of the convergence speed of hyperspectral image classification and classification accuracy needs to be improved, so as to improve the classification accuracy, the effect is obvious, The effect of high learning efficiency
- Summary
- Abstract
- Description
- Claims
- Application Information
AI Technical Summary
Problems solved by technology
Method used
Image
Examples
Embodiment
[0045] This embodiment provides a hyperspectral image active learning method based on image signal sampling, the process of the method is as follows figure 1 shown, including the following steps:
[0046] S1. Read the three-dimensional hyperspectral image data cube H(m,n,b), where m and n represent the spatial pixel position, and b represents the spectral band position;
[0047] S2. Rearrange the three-dimensional hyperspectral image data in step S1 into a two-dimensional matrix I(k,b) according to the order of pixel positions, wherein k represents the pixel label, k is an integer in the range of [1,V], and V is a pixel The total number of points, b represents the position of the spectral band;
[0048] S3. Use the hyperspectral image category label as the graph signal, and use the hyperspectral image data to calculate the correlation coefficient of the sample mean value between all pixels, and use it as the similarity connection relationship between the graph signal points t...
PUM
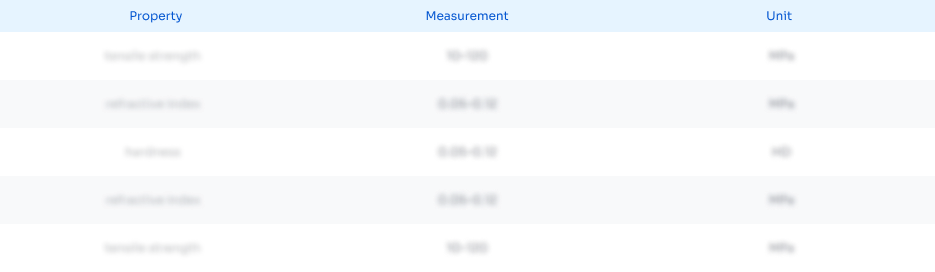
Abstract
Description
Claims
Application Information
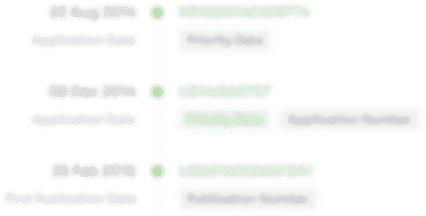
- R&D Engineer
- R&D Manager
- IP Professional
- Industry Leading Data Capabilities
- Powerful AI technology
- Patent DNA Extraction
Browse by: Latest US Patents, China's latest patents, Technical Efficacy Thesaurus, Application Domain, Technology Topic, Popular Technical Reports.
© 2024 PatSnap. All rights reserved.Legal|Privacy policy|Modern Slavery Act Transparency Statement|Sitemap|About US| Contact US: help@patsnap.com