A Fast Segmentation Method for Multimodal Surgical Trajectories Based on Unsupervised Deep Learning
A deep learning, unsupervised technology, applied in image analysis, character and pattern recognition, biological neural network model, etc., can solve the problems of insignificant video features, poor video feature quality, slow video feature extraction, etc., to reduce redundancy The effect of shifting points, improving feature quality, and speeding up extraction
- Summary
- Abstract
- Description
- Claims
- Application Information
AI Technical Summary
Problems solved by technology
Method used
Image
Examples
Embodiment
[0214] The data set used is the JIGSAWS data set published by Johns Hopkins University (Johns Hopkins University), which includes two parts: surgical data and manual annotation. The data set is collected from the da Vinci medical robot system and is divided into kinematic data and video data. The sampling frequency of both kinematic data and video data is 30Hz. The data set contains 3 tasks, needle threading (NP), suturing (SU) and knotting (KT), which are operated and annotated by doctors with different skill levels. In the experiment, it is found that the kinematics data of the data set has a small amount of segment trajectory noise and data jitter, so the kinematics data is smoothed by wavelet transform and then the trajectory is segmented.
[0215] A subset of the JIGSAWS data set is selected for verification, including two tasks of needle threading and suturing. Each surgical task contains 11 groups of demonstrations, from 5 experts (E), 3 intermediate experts (I), and 3...
PUM
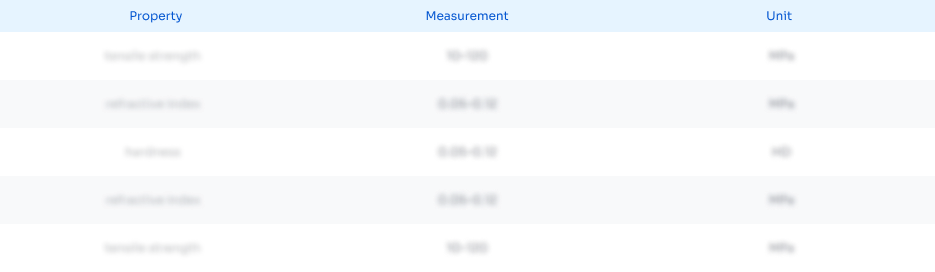
Abstract
Description
Claims
Application Information
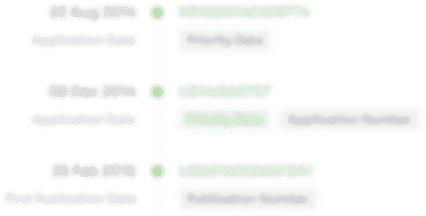
- R&D Engineer
- R&D Manager
- IP Professional
- Industry Leading Data Capabilities
- Powerful AI technology
- Patent DNA Extraction
Browse by: Latest US Patents, China's latest patents, Technical Efficacy Thesaurus, Application Domain, Technology Topic, Popular Technical Reports.
© 2024 PatSnap. All rights reserved.Legal|Privacy policy|Modern Slavery Act Transparency Statement|Sitemap|About US| Contact US: help@patsnap.com