Optimization of power system risk assessment method based on support vector machine using cross entropy theory
A support vector machine and power system technology, applied in the field of power system risk assessment based on cross-entropy theory optimization support vector machine, can solve problems such as local optimum, long training time, large deviation, etc., to reduce complexity and power consumption time, reduce the number of features, and test the effect of short time
- Summary
- Abstract
- Description
- Claims
- Application Information
AI Technical Summary
Problems solved by technology
Method used
Image
Examples
Embodiment Construction
[0023] Embodiments of the present invention will be described in detail below in conjunction with the accompanying drawings.
[0024] In view of the deficiencies of existing machine learning, the present embodiment applies the cross-entropy method (CEM) in the support vector machine (SVM) to perform feature selection and SVM parameter optimization, and uses the n-dimensional feature quantity constructed as the input of the SVM to utilize the training The samples are trained offline to generate a training model, and through the learning of historical samples, the risk level of the power system in the current state is predicted.
[0025] This embodiment is achieved through the following technical solutions:
[0026] The first part is to generate offline data, and use the SVM method of cross-entropy optimization to train the risk sample data to obtain the optimal characteristics and parameters of the power grid risk assessment model.
[0027] Step 1.1, select a power grid, colle...
PUM
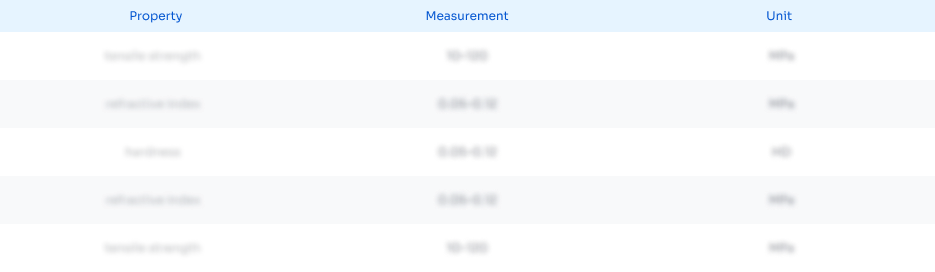
Abstract
Description
Claims
Application Information
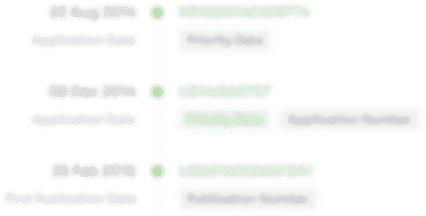
- R&D Engineer
- R&D Manager
- IP Professional
- Industry Leading Data Capabilities
- Powerful AI technology
- Patent DNA Extraction
Browse by: Latest US Patents, China's latest patents, Technical Efficacy Thesaurus, Application Domain, Technology Topic, Popular Technical Reports.
© 2024 PatSnap. All rights reserved.Legal|Privacy policy|Modern Slavery Act Transparency Statement|Sitemap|About US| Contact US: help@patsnap.com