Optimization method for signal timing in single-point crossroad based on deep reinforcement learning
A technology of reinforcement learning and single-point intersection, applied in the field of intelligent transportation, can solve problems such as poor robustness, inapplicability, and complex and cumbersome calculation processes, and achieve the effects of reducing traffic congestion, improving stability, and reducing complexity
- Summary
- Abstract
- Description
- Claims
- Application Information
AI Technical Summary
Problems solved by technology
Method used
Image
Examples
Embodiment Construction
[0024] The technical solution of the present invention will be further described in detail below in conjunction with the accompanying drawings, but the implementation and protection of the present invention are not limited to the content described below.
[0025] The invention is based on deep reinforcement learning, uses traffic simulation software SUMO to perform simulation iterations, and finally realizes self-adaptive timing optimization of single-point intersections. The specific implementation steps are as follows:
[0026] Step 1: Establish a single-point intersection through the SUMO simulation software. Here, a one-way three-lane intersection is established according to the actual road. like figure 2 As shown, the innermost lane is the left-turn lane, the middle lane is the through lane, and the right lane is the through and right-turn lane. After the intersection is established, enter the traffic volume of each lane for the four roads entering the intersection. Th...
PUM
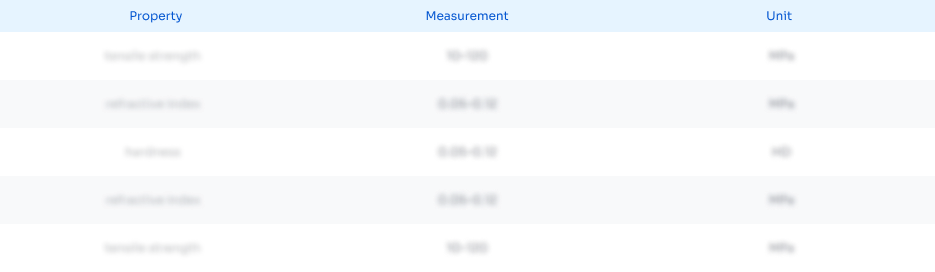
Abstract
Description
Claims
Application Information
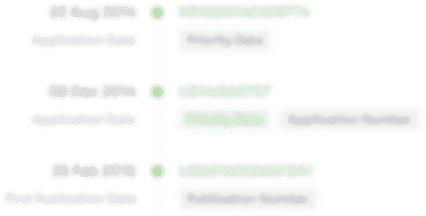
- R&D
- Intellectual Property
- Life Sciences
- Materials
- Tech Scout
- Unparalleled Data Quality
- Higher Quality Content
- 60% Fewer Hallucinations
Browse by: Latest US Patents, China's latest patents, Technical Efficacy Thesaurus, Application Domain, Technology Topic, Popular Technical Reports.
© 2025 PatSnap. All rights reserved.Legal|Privacy policy|Modern Slavery Act Transparency Statement|Sitemap|About US| Contact US: help@patsnap.com