A cross-modal retrieval method based on cyclic generation antagonistic network
A cross-modal, generative technology, applied in biological neural network models, neural learning methods, and other database retrievals, can solve problems such as retention, dependence, and inability to achieve cross-modal retrieval of unlabeled data to improve efficiency , Improve the effect of accuracy and stability
- Summary
- Abstract
- Description
- Claims
- Application Information
AI Technical Summary
Problems solved by technology
Method used
Image
Examples
Embodiment Construction
[0052] Below in conjunction with accompanying drawing and specific embodiment the present invention will be described in further detail:
[0053]In recent years, with the upsurge of artificial intelligence, deep learning technology has gradually emerged and affected many fields of computer science. In the field of multimedia information retrieval technology, more and more people use deep learning to improve the accuracy and accuracy of existing retrievals. stability. The generative adversarial network (generative adversarial network) used in this method is a new type of neural network that has been widely used in recent years to estimate the generative model through the confrontation process. The generator (generator) and The discriminator used to distinguish the authenticity of the data, the generator and the discriminator compete with each other during the training process, and finally reach a dynamic balance. Generative adversarial networks are widely used in many fields s...
PUM
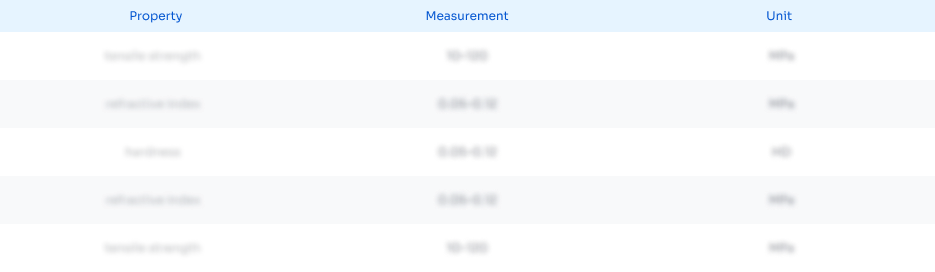
Abstract
Description
Claims
Application Information
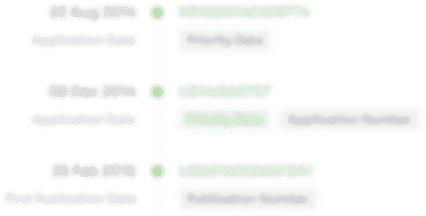
- Generate Ideas
- Intellectual Property
- Life Sciences
- Materials
- Tech Scout
- Unparalleled Data Quality
- Higher Quality Content
- 60% Fewer Hallucinations
Browse by: Latest US Patents, China's latest patents, Technical Efficacy Thesaurus, Application Domain, Technology Topic, Popular Technical Reports.
© 2025 PatSnap. All rights reserved.Legal|Privacy policy|Modern Slavery Act Transparency Statement|Sitemap|About US| Contact US: help@patsnap.com