A Pedestrian Trajectory Prediction Method Combining Social Force Model and Kalman Filter
A social force model and Kalman filter technology, applied in the field of pedestrian trajectory prediction, can solve the error of trajectory prediction results, without considering the relationship between pedestrians and pedestrians, pedestrians and obstacles, etc., to achieve lower errors and meet prediction requirements Effect
- Summary
- Abstract
- Description
- Claims
- Application Information
AI Technical Summary
Problems solved by technology
Method used
Image
Examples
Embodiment Construction
[0035] The present invention will be further described below.
[0036] Concrete steps of the present invention are:
[0037] 1. Kalman filter initialization;
[0038] In the tracking process, because the sampling time is very short, the movement of the target within the sampling time can be regarded as uniform motion. Therefore, the motion equation of the pedestrian is obtained as
[0039]
[0040] because
[0041] x k =AX k-1 +W k-1 (1)
[0042] Z k =HX k +V k (2)
[0043] In the formula, X k is the system state at time k, A is an n×n-dimensional state transition matrix; H is an m×n-dimensional observation matrix; W k-1 Gaussian distribution with mean zero and covariance matrix Q; V k To measure the noise, satisfy the Gaussian distribution whose covariance matrix is R;
[0044] Writing formula (7) in matrix form, the state transition matrix of the system can be obtained as: system state variables The measurement value extracted in the measurement upda...
PUM
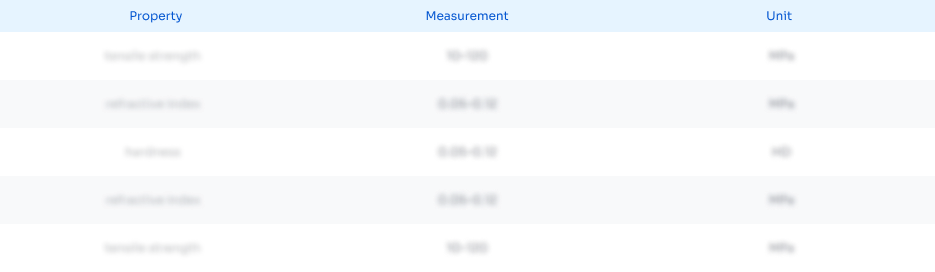
Abstract
Description
Claims
Application Information
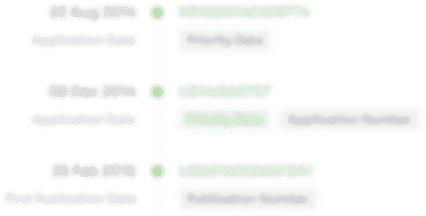
- R&D Engineer
- R&D Manager
- IP Professional
- Industry Leading Data Capabilities
- Powerful AI technology
- Patent DNA Extraction
Browse by: Latest US Patents, China's latest patents, Technical Efficacy Thesaurus, Application Domain, Technology Topic, Popular Technical Reports.
© 2024 PatSnap. All rights reserved.Legal|Privacy policy|Modern Slavery Act Transparency Statement|Sitemap|About US| Contact US: help@patsnap.com