Discovery method and system suitable for a plurality of hidden confounding factor data
A discovery method and factor technology, applied in the field of causal network discovery methods and systems, can solve problems such as high complexity, inability to effectively identify hidden confounding factors, inability to discover hidden confounding factors and observation variable relationships, etc.
- Summary
- Abstract
- Description
- Claims
- Application Information
AI Technical Summary
Problems solved by technology
Method used
Image
Examples
Embodiment Construction
[0056] The specific embodiment of the present invention will be further described below in conjunction with accompanying drawing:
[0057] Such as figure 1 As shown, a causal network discovery method suitable for data with multiple hidden confounding factors, including the following steps:
[0058] S1), setting observation data set X=[x 1 ,x 2 ,...,x N ], where each variable x i Include p sample data, and assume that there are unknown dimensions and the same number of hidden confounding factor sets Z=[z 1 ,z 2 ,…,z M ], and set the front causal order set K head , and the tail causal order set K tail is an empty set, the record contains the candidate variable set U={x of the observation data set 1 ,x 2 ,...,x N};
[0059] S2), starting from a completely undirected graph, using the method of (conditional) independence test to learn the causal skeleton graph G between the observed data, and record each observed variable x i The adjacent variable Adj(G,x i );
[006...
PUM
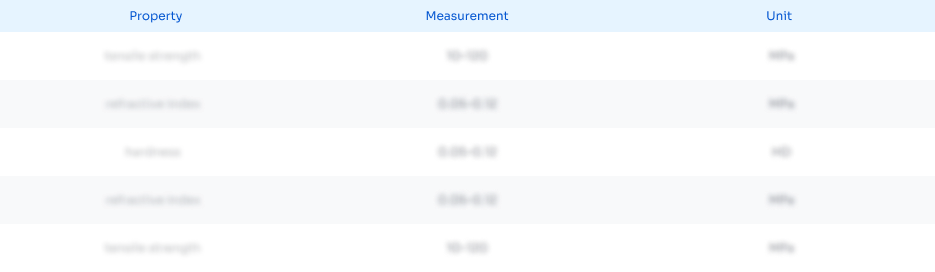
Abstract
Description
Claims
Application Information
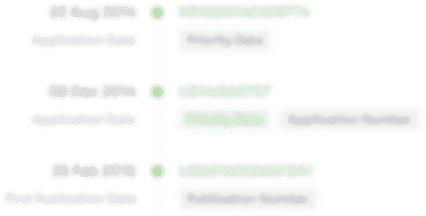
- R&D Engineer
- R&D Manager
- IP Professional
- Industry Leading Data Capabilities
- Powerful AI technology
- Patent DNA Extraction
Browse by: Latest US Patents, China's latest patents, Technical Efficacy Thesaurus, Application Domain, Technology Topic, Popular Technical Reports.
© 2024 PatSnap. All rights reserved.Legal|Privacy policy|Modern Slavery Act Transparency Statement|Sitemap|About US| Contact US: help@patsnap.com