Dynamic social network community evolution analysis method and system
A technology of social networking and analysis methods, applied in instruments, data processing applications, computing, etc., can solve the problems of distinguishing core node types, failing to make full use of network topology information, etc., to achieve high accuracy and efficiency, and reasonable division results , the effect of high execution efficiency
- Summary
- Abstract
- Description
- Claims
- Application Information
AI Technical Summary
Problems solved by technology
Method used
Image
Examples
Embodiment 1
[0086] A specific embodiment of the present invention discloses a method for analyzing the evolution of a dynamic social network community, such as figure 1 shown, including the following steps:
[0087] S1. For a given dynamic social network, starting from the first time slice, divide the community structure corresponding to the time slice network for each time slice;
[0088] S2. Calculate the superspreader set of each time slice network and the superblocker set of each community corresponding to the time slice network according to the community structure division results;
[0089] S3. For the above-mentioned superspreader set, determine the evolution event 1 type of each community; the possible types of the evolution event 1 include generation, merger and expansion events;
[0090] S4. For the above superblocker set, determine the evolution event 2 type of each community; the possible types of the evolution event 2 include disappearance, splitting and shrinking events.
...
Embodiment 2
[0093] Optimizing on the basis of Example 1, such as figure 2 As shown, step S1 can be further refined into the following steps:
[0094] S11. For a given dynamic social network, starting from the first time slice, obtain the neighbor relationship between each node in each time slice network;
[0095] S12. According to the neighbor relationship above, divide the community structure corresponding to each time slice network through the QCA algorithm.
[0096] The QCA algorithm is a fast adaptive dynamic community discovery algorithm based on modularity. It formulates different community structure update strategies for each network change, including node addition, node deletion, edge addition, and edge deletion. By maximizing modularity To determine the community affiliation of incremental nodes.
[0097] Preferably, as image 3 As shown, step S2 can be further refined into the following steps:
[0098] S21. Obtain the superspreader set of each time slice network through the...
Embodiment 3
[0145] Another specific embodiment of the present invention discloses a dynamic social network community evolution analysis system, which uses the method described in Embodiment 1 to perform evolution analysis. Specifically, such as Image 6 As shown, the dynamic social network community evolution analysis system includes a community division module, a superspreader set calculation module, a superblocker set calculation module, a superspreader-based evolution event analysis module, and a superblocker-based evolution event analysis module. Among them, the output of the community division module is connected to the input of the superspreader set calculation module, the superblocker set calculation module, the superspreader-based evolution event analysis module, and the superblocker-based evolution event analysis module. The output end of the superspreader set calculation module is connected to the input end of the superspreader-based evolution event analysis module, and the outp...
PUM
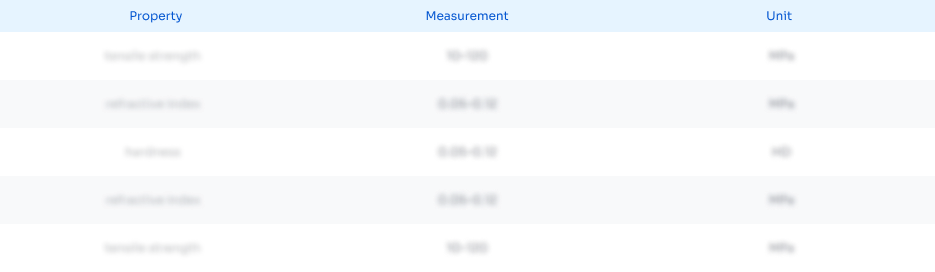
Abstract
Description
Claims
Application Information
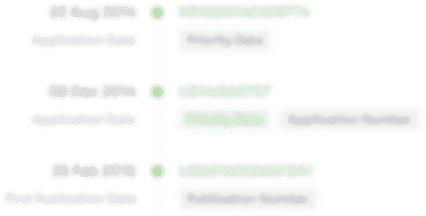
- Generate Ideas
- Intellectual Property
- Life Sciences
- Materials
- Tech Scout
- Unparalleled Data Quality
- Higher Quality Content
- 60% Fewer Hallucinations
Browse by: Latest US Patents, China's latest patents, Technical Efficacy Thesaurus, Application Domain, Technology Topic, Popular Technical Reports.
© 2025 PatSnap. All rights reserved.Legal|Privacy policy|Modern Slavery Act Transparency Statement|Sitemap|About US| Contact US: help@patsnap.com