Load forecasting method and device based on neural network
A technology of load forecasting and neural network, applied in the field of communication, can solve problems such as large forecast deviation, non-stationary sequence cannot be found, unfavorable scheduling optimization, etc., and achieve the effect of reducing forecast deviation and improving accuracy
- Summary
- Abstract
- Description
- Claims
- Application Information
AI Technical Summary
Problems solved by technology
Method used
Image
Examples
Embodiment 1
[0061] The method embodiment provided in Embodiment 1 of the present application may be executed in a server, a network terminal, a computer terminal or a similar computing device. Take running on a network terminal as an example, figure 1It is a block diagram of the hardware structure of a neural network-based load forecasting network terminal according to an embodiment of the present invention. like figure 1 As shown, the network terminal 10 may include one or more ( figure 1 Only one is shown in the figure) a processor 102 (the processor 102 may include but not limited to a processing device such as a microprocessor MCU or a programmable logic device FPGA) and a memory 104 for storing data. Optionally, the above-mentioned network terminal also A transmission device 106 for communication functions as well as input and output devices 108 may be included. Those of ordinary skill in the art can understand that, figure 1 The shown structure is only for illustration, and does...
Embodiment 2
[0119] In this embodiment, a neural network-based load forecasting device is also provided, which is used to implement the above-mentioned embodiments and preferred implementation modes, and those that have already been described will not be repeated. As used below, the term "module" may be a combination of software and / or hardware that realizes a predetermined function. Although the devices described in the following embodiments are preferably implemented in software, implementations in hardware, or a combination of software and hardware are also possible and contemplated.
[0120] image 3 is a structural block diagram of a neural network-based load forecasting device according to an embodiment of the present invention, such as image 3 As shown, the device includes:
[0121] A receiving module 30, configured to receive a time period to be predicted;
[0122] The input module 32 is used to input the time period into a neural network model for predicting energy load, where...
Embodiment 3
[0127] An embodiment of the present invention also provides a storage medium, in which a computer program is stored, wherein the computer program is set to execute the steps in any one of the above method embodiments when running.
[0128] Optionally, in this embodiment, the above-mentioned storage medium may be configured to store a computer program for performing the following steps:
[0129] S1, receiving the time period to be predicted;
[0130] S2, inputting the time period into a neural network model for predicting energy load, wherein the neural network model is composed of a linear prediction model and a nonlinear prediction model;
[0131] S3. Using the neural network model to predict the energy load value in the time period.
[0132] Optionally, in this embodiment, the above-mentioned storage medium may include but not limited to: U disk, read-only memory (Read-Only Memory, ROM for short), random access memory (Random Access Memory, RAM for short), Various media th...
PUM
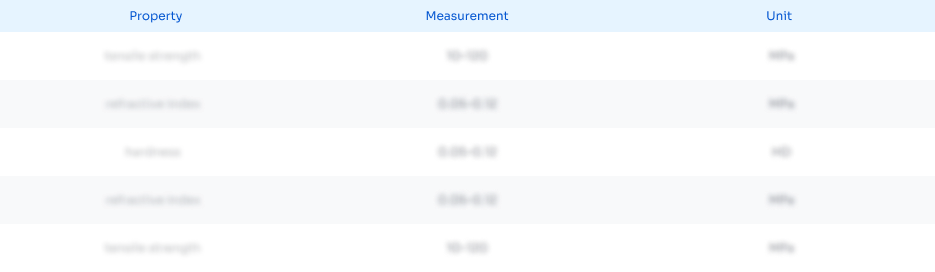
Abstract
Description
Claims
Application Information
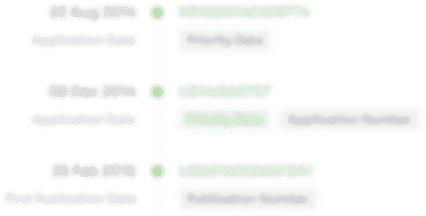
- R&D Engineer
- R&D Manager
- IP Professional
- Industry Leading Data Capabilities
- Powerful AI technology
- Patent DNA Extraction
Browse by: Latest US Patents, China's latest patents, Technical Efficacy Thesaurus, Application Domain, Technology Topic, Popular Technical Reports.
© 2024 PatSnap. All rights reserved.Legal|Privacy policy|Modern Slavery Act Transparency Statement|Sitemap|About US| Contact US: help@patsnap.com