A nuclear magnetic resonance multi-weighted imaging method based on a depth generative adversarial neural network
A technology of nuclear magnetic resonance images and neural networks, applied in biological neural network models, neural learning methods, neural architectures, etc., to achieve the effect of reducing discomfort and medical expenses
- Summary
- Abstract
- Description
- Claims
- Application Information
AI Technical Summary
Problems solved by technology
Method used
Image
Examples
Embodiment Construction
[0060] Below in conjunction with accompanying drawing, the present invention will be further described, a kind of nuclear magnetic resonance multi-weighted imaging method based on deep generation confrontational neural network is as follows figure 1 shown, including the following steps:
[0061] 1) Construct a deep generative confrontational neural network, including the following steps:
[0062] 1.1) Construct the generation network, that is, the generation network includes a batch normalization layer, a convolution layer, a deconvolution layer and a fully connected layer, wherein the batch normalization output of the convolution layer and the batch normalization of the corresponding deconvolution layer The output is skipped and added through the fully connected layer as the input of the next deconvolution layer, such as image 3 shown;
[0063] 1.2) Construct discriminant network, discriminant network is used for the probability that output image is judged to be PD weighte...
PUM
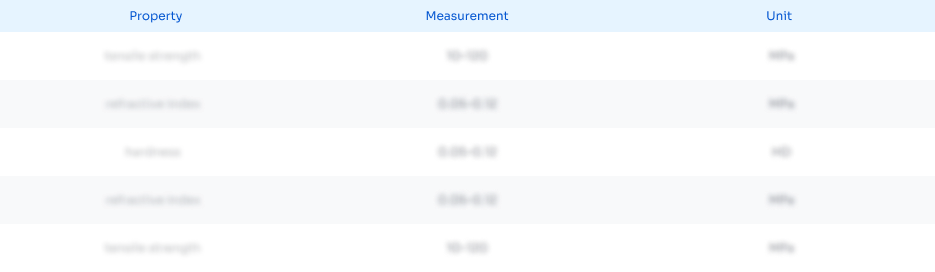
Abstract
Description
Claims
Application Information
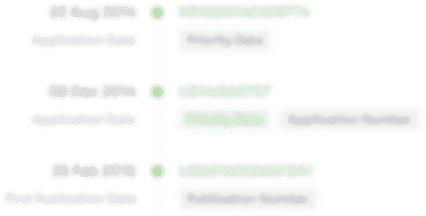
- R&D Engineer
- R&D Manager
- IP Professional
- Industry Leading Data Capabilities
- Powerful AI technology
- Patent DNA Extraction
Browse by: Latest US Patents, China's latest patents, Technical Efficacy Thesaurus, Application Domain, Technology Topic.
© 2024 PatSnap. All rights reserved.Legal|Privacy policy|Modern Slavery Act Transparency Statement|Sitemap