An intelligent rotary machine fault depth network feature identification method
A rotating machinery and fault technology, applied in the field of intelligent rotating machinery fault deep network feature identification, can solve the problems of high training sample dimension, poor effect, general effect, etc.
- Summary
- Abstract
- Description
- Claims
- Application Information
AI Technical Summary
Problems solved by technology
Method used
Image
Examples
Embodiment Construction
[0067] The intelligent rotating machinery fault deep network feature identification method provided by the embodiment of the present invention collects the original vibration sequence of the rolling bearing when the rolling bearing is in operation by installing a vibration sensor on the part of the rotating machinery to be detected in the rolling bearing of the train, and then decomposes the original vibration sequence through the singular spectrum analysis method Reconstruct and extract the root mean square value, standard deviation, skewness index and peak value of the reconstructed vibration sequence, use the rotating machinery fault location diagnosis model trained by the support vector machine to judge the fault location, and then assemble the reconstructed vibration sequence Empirical mode decomposition calculates the permutation entropy values of a set of decomposed intrinsic modal components, uses the permutation and combination of permutation entropy values as detec...
PUM
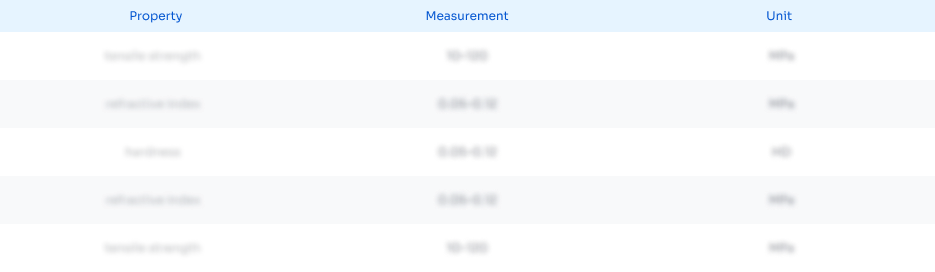
Abstract
Description
Claims
Application Information
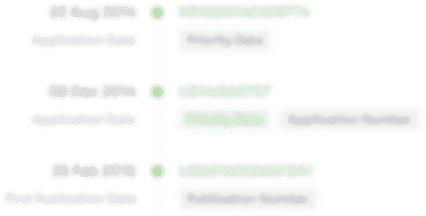
- R&D Engineer
- R&D Manager
- IP Professional
- Industry Leading Data Capabilities
- Powerful AI technology
- Patent DNA Extraction
Browse by: Latest US Patents, China's latest patents, Technical Efficacy Thesaurus, Application Domain, Technology Topic, Popular Technical Reports.
© 2024 PatSnap. All rights reserved.Legal|Privacy policy|Modern Slavery Act Transparency Statement|Sitemap|About US| Contact US: help@patsnap.com