Variational expectation maximization routing algorithm based on a capsule network
A technology of expectation maximization and capsules, which is applied in biological neural network models, neural learning methods, neural architectures, etc., and can solve non-computable problems
- Summary
- Abstract
- Description
- Claims
- Application Information
AI Technical Summary
Problems solved by technology
Method used
Image
Examples
Embodiment Construction
[0071] The present invention will be further described in detail below in conjunction with the accompanying drawings and through specific embodiments. The following embodiments are only descriptive, not restrictive, and cannot limit the protection scope of the present invention.
[0072] In order to achieve the purpose and effect of the technical means, creation features, work flow, and use method of the present invention, and to make the evaluation method easy to understand, the present invention will be further described below in conjunction with specific examples.
[0073] Such as figure 2 , image 3 As shown, the mnist data set is used as the training data of the capsule network. The original data size is 28*28. In the ordinary convolution layer, the convolution kernel is 5x5, the step size is 2, and the image is convolved with padding. Get 14*14*32 input;
[0074] In the initial capsule layer, a 1x1 convolution kernel is used to convert 32 channels into 32 primary caps...
PUM
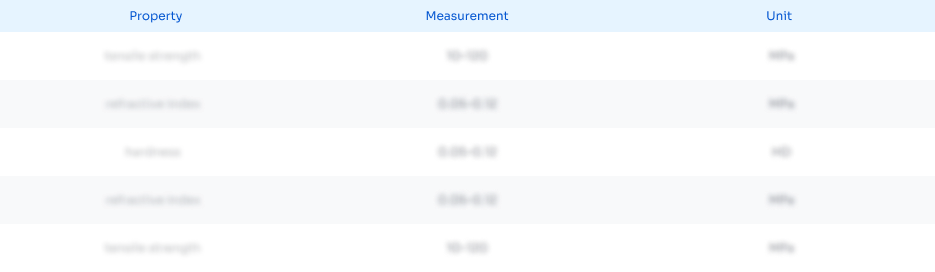
Abstract
Description
Claims
Application Information
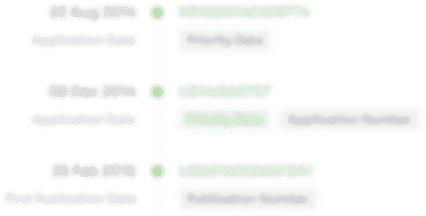
- Generate Ideas
- Intellectual Property
- Life Sciences
- Materials
- Tech Scout
- Unparalleled Data Quality
- Higher Quality Content
- 60% Fewer Hallucinations
Browse by: Latest US Patents, China's latest patents, Technical Efficacy Thesaurus, Application Domain, Technology Topic, Popular Technical Reports.
© 2025 PatSnap. All rights reserved.Legal|Privacy policy|Modern Slavery Act Transparency Statement|Sitemap|About US| Contact US: help@patsnap.com