Image generation method based on a conditional capsule generative adversarial network
An image generation and network technology, applied in the field of image processing, can solve the problems of reducing the scale of network parameters and not considering the important spatial levels of simple and complex objects.
- Summary
- Abstract
- Description
- Claims
- Application Information
AI Technical Summary
Problems solved by technology
Method used
Image
Examples
Embodiment
[0057] as attached figure 1 As shown, this embodiment discloses an image generation method based on conditional capsule generative adversarial networks. In this example, the MNIST handwriting data set will be used as experimental data, and the generation goal is to generate handwritten digits. Among them, the generative confrontation network includes a generator and a discriminator. Generator part: The generator receives random noise and conditional vectors as input data, and outputs the generated image; then discriminator part: The discriminator receives generated images, real images (MINST dataset images) and conditional vectors as input data, and outputs generated The similarity between the image and the real image; by training this model structure, the model parameters of the generator are finally retained, and the handwritten digits can be generated by using the model parameters of the generator. The method for generating includes the following steps:
[0058] The exper...
PUM
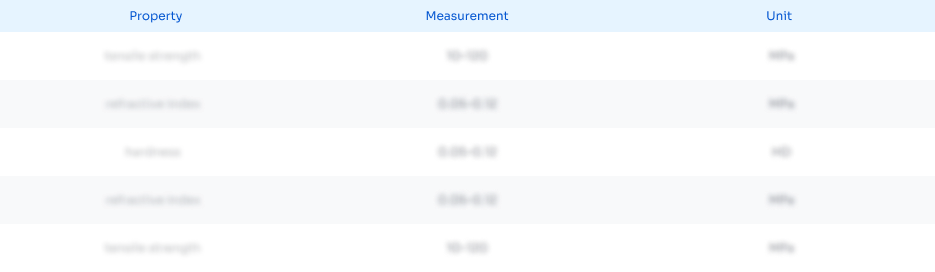
Abstract
Description
Claims
Application Information
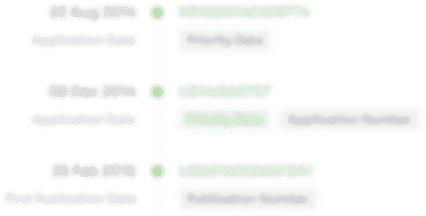
- R&D Engineer
- R&D Manager
- IP Professional
- Industry Leading Data Capabilities
- Powerful AI technology
- Patent DNA Extraction
Browse by: Latest US Patents, China's latest patents, Technical Efficacy Thesaurus, Application Domain, Technology Topic, Popular Technical Reports.
© 2024 PatSnap. All rights reserved.Legal|Privacy policy|Modern Slavery Act Transparency Statement|Sitemap|About US| Contact US: help@patsnap.com