Video content semantic understanding method based on a recurrent convolutional neural network
A neural network and recursive convolution technology, applied in the field of computer vision, can solve problems such as loss of information, dimensionality disaster, and poor robustness of scene switching, and achieve accurate recognition results, fast calculation speed, and small space occupation.
- Summary
- Abstract
- Description
- Claims
- Application Information
AI Technical Summary
Problems solved by technology
Method used
Image
Examples
Embodiment 1
[0043] A method of semantic understanding of video content based on recursive convolutional neural network. Recursive convolutional neural network is the model, such as figure 1 As shown, the convolutional neural network is used as the core of the recurrent neural network. In this method, the initial frame of the video is input to the recurrent neural network, and the initial variable that represents the initial state of the video is connected according to the depth dimension of the picture. The network uses a convolutional neural network for feature extraction, and the obtained feature output is used as the new hidden layer data to characterize the video state, which is passed to the next time step, and the above operation is repeated. On this basis, the hidden layer state of the recurrent neural network is used as the output and provided to the fully connected neural network classifier. After the feature reorganization of the fully connected classifier, the category output of t...
Embodiment 2
[0057] According to the method for semantic understanding of video content based on recursive convolutional neural network described in embodiment 1, the difference lies in:
[0058] In step (3), after the recursive convolutional neural network inputs a certain frame of video data, combined with the state data passed at the previous moment, the feature extraction on the current frame is performed, as shown in formula (I):
[0059] Ht+1=C{Ht: F t+1 } (Ⅰ)
[0060] In formula (Ⅰ), F t+1 Represents the t+1 frame data of the video, Ht is the video state represented by the hidden layer state of the previous time step, and C represents the convolution operation;
[0061] Step (5): After the final output of the sixth layer of the recursive convolutional neural network passes through the neural network classifier, the probability distribution of the data in each action category is calculated through the softmax operation, as shown in formula (II):
[0062] Prediction=softmax{W·H n } (Ⅱ)
[0063] ...
PUM
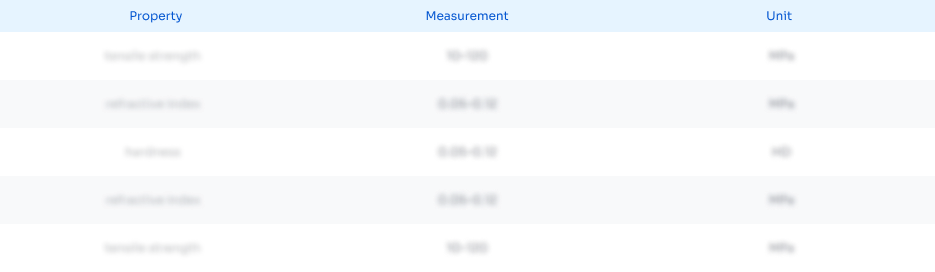
Abstract
Description
Claims
Application Information
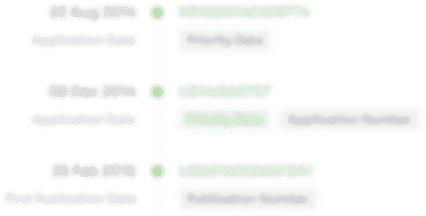
- Generate Ideas
- Intellectual Property
- Life Sciences
- Materials
- Tech Scout
- Unparalleled Data Quality
- Higher Quality Content
- 60% Fewer Hallucinations
Browse by: Latest US Patents, China's latest patents, Technical Efficacy Thesaurus, Application Domain, Technology Topic, Popular Technical Reports.
© 2025 PatSnap. All rights reserved.Legal|Privacy policy|Modern Slavery Act Transparency Statement|Sitemap|About US| Contact US: help@patsnap.com