An integrated circuit for epilepsy detection based on sparse extreme learning machine algorithm
A technology of extreme learning machine and integrated circuit, applied in computing, medical science, computer components, etc.
- Summary
- Abstract
- Description
- Claims
- Application Information
AI Technical Summary
Problems solved by technology
Method used
Image
Examples
Embodiment Construction
[0069] The present invention provides an epilepsy detection integrated circuit based on the algorithm of sparse extreme learning machine, which first generates a four-dimensional signal through the wavelet transform circuit module of the known type of EEG signal, and then calculates the circuit and mean value through the standard deviation in the feature extraction circuit module The calculation circuit generates a set of eight-dimensional vectors for each signal extraction feature, and then inputs these feature vectors to the classifier circuit module for training, and finally the EEG signals of unknown categories pass through the wavelet transform circuit module and feature extraction module, and then input to the classification Classify circuit modules. The invention can classify the EEG signals of epileptic seizures and non-epileptic seizures, so as to achieve the purpose of epilepsy detection.
[0070] see figure 1 , the present invention is an epilepsy detection integra...
PUM
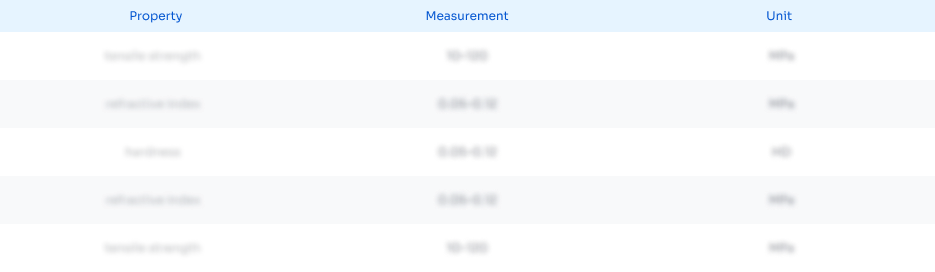
Abstract
Description
Claims
Application Information
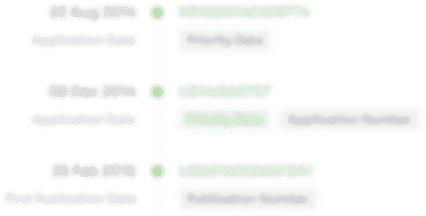
- R&D Engineer
- R&D Manager
- IP Professional
- Industry Leading Data Capabilities
- Powerful AI technology
- Patent DNA Extraction
Browse by: Latest US Patents, China's latest patents, Technical Efficacy Thesaurus, Application Domain, Technology Topic, Popular Technical Reports.
© 2024 PatSnap. All rights reserved.Legal|Privacy policy|Modern Slavery Act Transparency Statement|Sitemap|About US| Contact US: help@patsnap.com