A microgrid load prediction method based on an LSSVM neural network
A load forecasting and neural network technology, applied in neural learning methods, biological neural network models, forecasting, etc., can solve problems such as increased forecasting errors, low forecasting accuracy, and uneven load characteristic curves, so as to improve accuracy, learn High efficiency and global optimal effect
- Summary
- Abstract
- Description
- Claims
- Application Information
AI Technical Summary
Problems solved by technology
Method used
Image
Examples
Embodiment 1
[0034] Embodiment 1: a kind of microgrid load forecasting method based on the neural network of LSSVM, comprises the following steps:
[0035] Step 1 normalizes and preprocesses the historical data of the microgrid to form a training sample set; since the dimension and magnitude of the characteristic indexes are not the same, the effect of a characteristic index with a particularly large magnitude on the classification may be highlighted during the calculation process. In order to eliminate the influence of the difference in characteristic index units and the different magnitudes of characteristic indexes, it must be normalized so that each index value is unified in a certain common numerical characteristic range.
[0036] (1) Normalization of load data
[0037] Apply logarithmic treatment to the loadings:
[0038] x' ab = lg(x ab ) (2.1)
[0039] where x ab is the original load, x′ ab is the normalized load.
[0040] (2) Division and standardization of day types
[00...
PUM
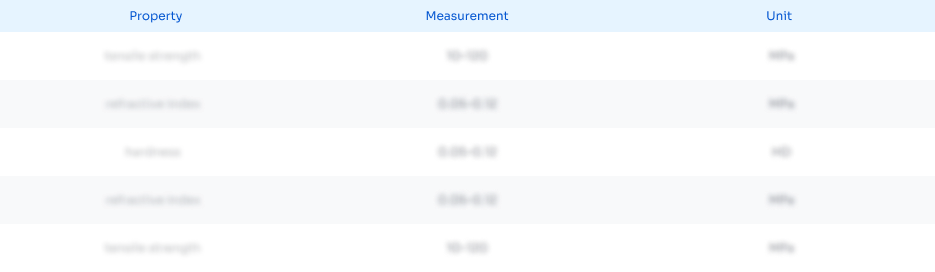
Abstract
Description
Claims
Application Information
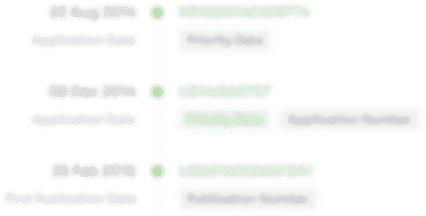
- R&D
- Intellectual Property
- Life Sciences
- Materials
- Tech Scout
- Unparalleled Data Quality
- Higher Quality Content
- 60% Fewer Hallucinations
Browse by: Latest US Patents, China's latest patents, Technical Efficacy Thesaurus, Application Domain, Technology Topic, Popular Technical Reports.
© 2025 PatSnap. All rights reserved.Legal|Privacy policy|Modern Slavery Act Transparency Statement|Sitemap|About US| Contact US: help@patsnap.com