A method for detecting red beetles based on deep learning
A deep learning, silverfish technology, applied in the direction of instrument, calculation, character and pattern recognition, etc., can solve the problems of high cost, strong subjectivity, high labor intensity, etc.
- Summary
- Abstract
- Description
- Claims
- Application Information
AI Technical Summary
Problems solved by technology
Method used
Image
Examples
Embodiment Construction
[0036] In recent years, deep learning has performed well in the field of target detection, which can simultaneously realize target positioning and recognition. The current relatively successful deep learning target detection networks include Faster R-CNN, SSD (Single Shot Multibox Detector), RetinaNet, Mask R-CNN, etc. in:
[0037] Faster R-CNN is a Region-Based NeuralNetwork based on RPN (region proposal network), which is a typical two-stage model. In the first stage of the image, a 2-class RPN is used to extract its region of interest (RoI), and in the second stage, Fast R-CNN is used to perform refine operations such as subdivision and position regression of the region of interest.
[0038] SSD is a typical single-stage model. An additional network structure is added after the truncated basic network, and feature maps with different resolutions are generated step by step (the deeper the network layer, the lower the resolution, and the stronger the semantic interpretation ...
PUM
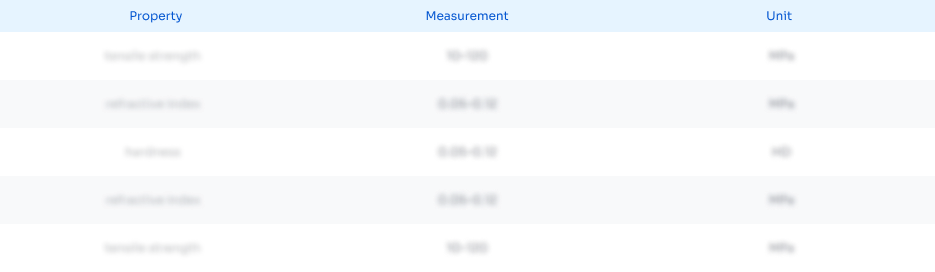
Abstract
Description
Claims
Application Information
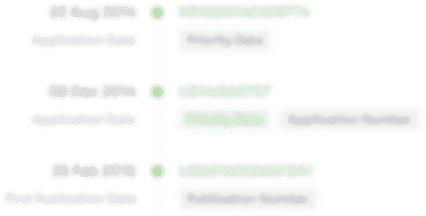
- R&D
- Intellectual Property
- Life Sciences
- Materials
- Tech Scout
- Unparalleled Data Quality
- Higher Quality Content
- 60% Fewer Hallucinations
Browse by: Latest US Patents, China's latest patents, Technical Efficacy Thesaurus, Application Domain, Technology Topic, Popular Technical Reports.
© 2025 PatSnap. All rights reserved.Legal|Privacy policy|Modern Slavery Act Transparency Statement|Sitemap|About US| Contact US: help@patsnap.com