A credit risk monitoring method integrating a deep belief network and an isolated forest algorithm
A technology of deep belief network and forest algorithm, applied in the field of computer software, can solve the problems of less related research and achieve the effect of improving classification performance
- Summary
- Abstract
- Description
- Claims
- Application Information
AI Technical Summary
Problems solved by technology
Method used
Image
Examples
Embodiment Construction
[0020] The invention uses a deep belief network to perform feature learning on high-dimensional data, obtains dimensionally reduced data as an input of an isolated forest algorithm, and improves the classification performance of the isolated forest algorithm for abnormal detection on high-dimensional data.
[0021] figure 1 It is a scheme diagram of the isolation forest algorithm classification method integrated with the deep belief network. First, data cleaning, missing value filling and data normalization are performed on the original financial transaction data, and finally the required data set is randomly sampled for the experiment, and finally standardized training subsets and test subsets are formed. The second part is the DBN data dimensionality reduction part and the optimized isolation forest algorithm, namely step 2, step 3 and step 4. The implementation of these two parts will be introduced in detail below.
[0022] Regarding step 2, construct a deep belief network...
PUM
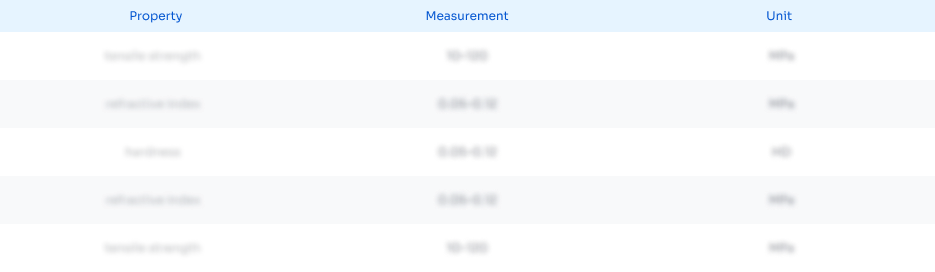
Abstract
Description
Claims
Application Information
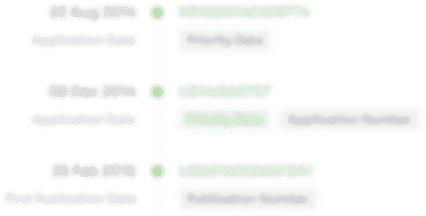
- R&D Engineer
- R&D Manager
- IP Professional
- Industry Leading Data Capabilities
- Powerful AI technology
- Patent DNA Extraction
Browse by: Latest US Patents, China's latest patents, Technical Efficacy Thesaurus, Application Domain, Technology Topic, Popular Technical Reports.
© 2024 PatSnap. All rights reserved.Legal|Privacy policy|Modern Slavery Act Transparency Statement|Sitemap|About US| Contact US: help@patsnap.com