CNN (Convolutional Neural Network)-based voiceprint recognition method for anti-record attack detection
A convolutional neural network and neural network technology, applied in the field of voiceprint authentication for anti-recording attack detection, can solve the problem of large model consumption and achieve the effect of reducing calculation and model size
- Summary
- Abstract
- Description
- Claims
- Application Information
AI Technical Summary
Problems solved by technology
Method used
Image
Examples
Embodiment 1
[0025] figure 1 As shown, the voiceprint authentication method based on the convolutional neural network proposed in this embodiment mainly includes:
[0026] Step 101: Acquire the audio to be detected, perform pre-emphasis processing and endpoint detection, and extract the MFCC feature vector of the audio to be detected. The audio to be detected includes the real voice of a person and the sound played after recording by different recording devices.
[0027] Step 102: Combining the deep decomposable operation of MobileNet and the way of connecting the first layer and the last layer neural network of Unet to construct a new convolutional neural network; in the network structure, the input layer is connected to a standard convolutional layer, and then used Four layers of downsampling convolutional layers with a step size of 2, and then four layers of upsampling deconvolution layers with a step size of 2. The first layer of convolutional layer is directly connected to the last la...
Embodiment 2
[0030] figure 2 As shown, the voiceprint authentication method based on the convolutional neural network in this embodiment to prevent recording attacks mainly includes:
[0031] Step 201: Acquire the audio to be detected, perform pre-emphasis processing and endpoint detection, and extract the MFCC feature vector of the audio to be detected. The audio to be detected includes the real voice of a person and the sound recorded and played by different recording devices.
[0032] Step 202: Using the feature vector proposed in step S101, train a fully connected neural network, the input and output of the model are the MFCC features extracted in S101, that is, train an autoencoder.
[0033] Step 203: pass all the audio through the fully connected neural network trained in step 202, and take the output of the bottleneck layer as the feature input of the new network.
[0034] Step 204: Combining the deep decomposable operation of MobileNet and the way of connecting the first layer an...
PUM
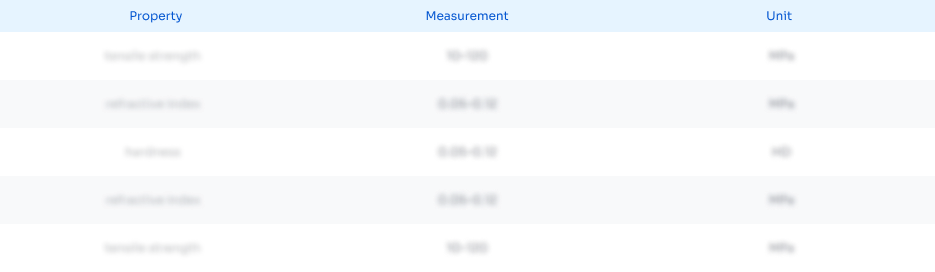
Abstract
Description
Claims
Application Information
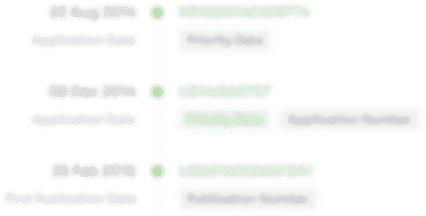
- R&D
- Intellectual Property
- Life Sciences
- Materials
- Tech Scout
- Unparalleled Data Quality
- Higher Quality Content
- 60% Fewer Hallucinations
Browse by: Latest US Patents, China's latest patents, Technical Efficacy Thesaurus, Application Domain, Technology Topic, Popular Technical Reports.
© 2025 PatSnap. All rights reserved.Legal|Privacy policy|Modern Slavery Act Transparency Statement|Sitemap|About US| Contact US: help@patsnap.com