A new project collaborative recommendation method based on multi-core fusion
A multi-core fusion and recommendation method technology, applied in the field of machine learning, can solve the problems of difficulty in effectively matching similarity and user preferences, poor algorithm adaptability, low reliability, etc., to improve accuracy and feasibility. , the effect of simplifying complexity
- Summary
- Abstract
- Description
- Claims
- Application Information
AI Technical Summary
Problems solved by technology
Method used
Image
Examples
Embodiment Construction
[0054] The present invention provides an embodiment of a new project collaborative recommendation method based on multi-core fusion, in order to enable those skilled in the art to better understand the technical solutions in the embodiments of the present invention, and to make the above-mentioned purposes, features and advantages of the present invention It can be more obvious and understandable, and the technical solution in the present invention will be described in further detail below in conjunction with the accompanying drawings:
[0055] The present invention provides an embodiment of a new item collaborative recommendation method based on multi-core fusion, such as figure 1 shown, including:
[0056] S101. Step 1. Establishing a data attribute information set, the data attribute information set including: user ID information, item ID information, scoring information, and item attribute information;
[0057]S102, step 2, extracting user ID information and project ID in...
PUM
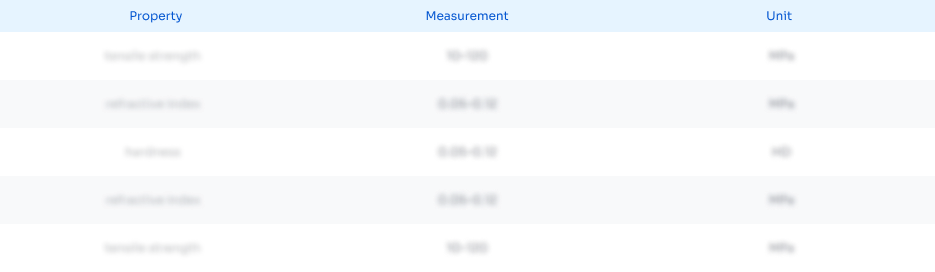
Abstract
Description
Claims
Application Information
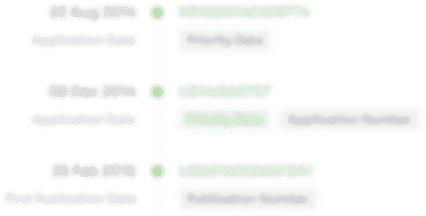
- Generate Ideas
- Intellectual Property
- Life Sciences
- Materials
- Tech Scout
- Unparalleled Data Quality
- Higher Quality Content
- 60% Fewer Hallucinations
Browse by: Latest US Patents, China's latest patents, Technical Efficacy Thesaurus, Application Domain, Technology Topic, Popular Technical Reports.
© 2025 PatSnap. All rights reserved.Legal|Privacy policy|Modern Slavery Act Transparency Statement|Sitemap|About US| Contact US: help@patsnap.com