Deep neural network magnetic resonance signal denoising method based on discrete cosine transform
A technology of discrete cosine transform and deep neural network, applied in electron magnetic resonance/nuclear magnetic resonance detection, acoustic re-radiation, electric/magnetic exploration, etc., can solve problems such as the complexity of the denoising method
- Summary
- Abstract
- Description
- Claims
- Application Information
AI Technical Summary
Problems solved by technology
Method used
Image
Examples
Embodiment
[0096] A deep neural network magnetic resonance signal denoising method based on discrete cosine transform, comprising the following steps:
[0097] A, add air mining nuclear magnetic resonance noise in the simulated nuclear magnetic resonance signal, do discrete cosine transform DCT, obtain the training data set and test data set of neural network;
[0098] B. Perform mean normalization processing on the training data set and the test data set;
[0099] C. Set up the deep neural network structure, pre-train the DNN with the RBM training method, and obtain the initial network weight of the DNN;
[0100] D. Use the backpropagation algorithm to supervise the global training of DNN and fine-tune the weight parameters of the DNN network;
[0101] E. Input the test data set into the trained DNN, denormalize the output of the DNN, and perform inverse discrete cosine transform to obtain the denoised nuclear magnetic resonance time domain signal.
[0102] Described step A comprises th...
PUM
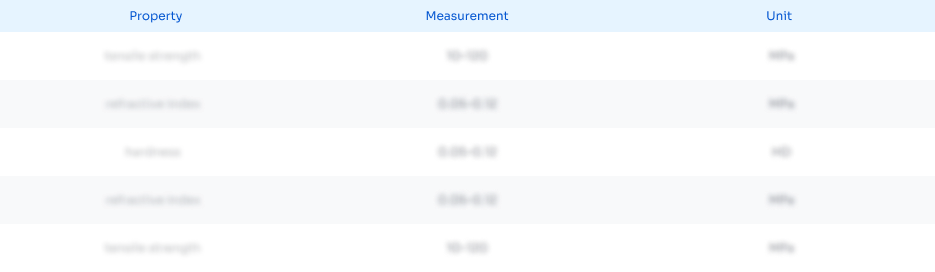
Abstract
Description
Claims
Application Information
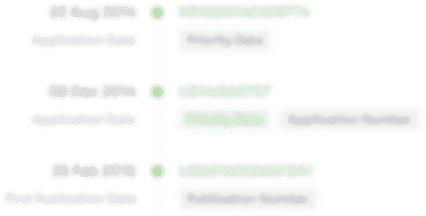
- R&D Engineer
- R&D Manager
- IP Professional
- Industry Leading Data Capabilities
- Powerful AI technology
- Patent DNA Extraction
Browse by: Latest US Patents, China's latest patents, Technical Efficacy Thesaurus, Application Domain, Technology Topic.
© 2024 PatSnap. All rights reserved.Legal|Privacy policy|Modern Slavery Act Transparency Statement|Sitemap