Gear Remaining Life Prediction Method Integrated with Kernel Estimation and Random Filtering
A random filtering and life prediction technology, which is applied in the testing of mechanical components, testing of machine/structural components, measuring devices, etc., can solve problems such as inability to guarantee global convergence, large gaps, and prediction models that cannot adapt to changes in the environment
- Summary
- Abstract
- Description
- Claims
- Application Information
AI Technical Summary
Problems solved by technology
Method used
Image
Examples
Embodiment Construction
[0056] Embodiment of the present invention will be further described below in conjunction with accompanying drawing:
[0057] Such as figure 2 The schematic diagram of the test bench for this test application is shown, and the steps of the test method are as follows:
[0058] Step 1. Obtain real-time monitoring data representing the state of the gears in the main test gearbox through the test bench:
[0059] use as figure 2 For the test bench shown, the center distance of the test bench is a=150mm. The test is loaded by mechanical lever 4, and the torque is measured by torque sensor 13#. During the test, the vibration acceleration, temperature and noise of the gear are monitored by receiving sensor signals. Such as image 3 As shown, the gear box 1 of the main test is a pair of gears with positive and negative overlapping meshing, and the state of broken teeth of the gears is equivalent to the failure of the gears. The test monitors the data of the main gear box 1. Durin...
PUM
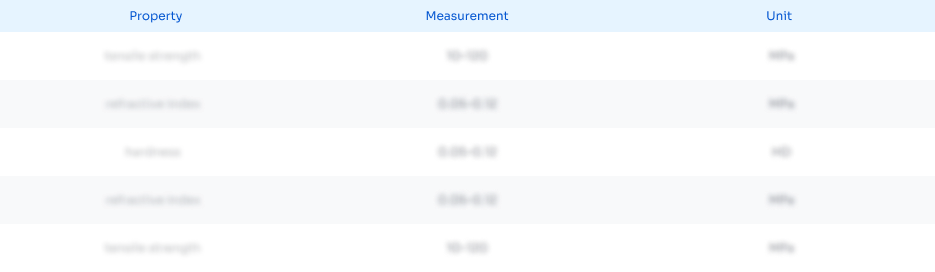
Abstract
Description
Claims
Application Information
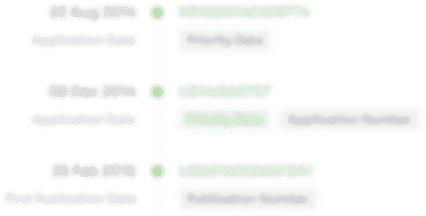
- R&D Engineer
- R&D Manager
- IP Professional
- Industry Leading Data Capabilities
- Powerful AI technology
- Patent DNA Extraction
Browse by: Latest US Patents, China's latest patents, Technical Efficacy Thesaurus, Application Domain, Technology Topic, Popular Technical Reports.
© 2024 PatSnap. All rights reserved.Legal|Privacy policy|Modern Slavery Act Transparency Statement|Sitemap|About US| Contact US: help@patsnap.com