An active learning and deep learning combined aluminum material surface defect detection method
A technology of active learning and deep learning, applied in neural learning methods, image data processing, instruments, etc., can solve problems such as unsatisfactory accuracy and insufficient models, and achieve the goal of improving accuracy, saving training data, and ensuring classification accuracy Effect
- Summary
- Abstract
- Description
- Claims
- Application Information
AI Technical Summary
Problems solved by technology
Method used
Image
Examples
Embodiment 1
[0027] An aluminum surface defect detection method combining active learning and deep learning, see figure 1 , the method includes the following steps:
[0028] 101: Obtain a data set, extract 80% of the data from each category of the data set as a training set, and use the remaining 20% of the data as a verification set;
[0029] 102: Perform data enhancement on the data in the training set by randomly adjusting image saturation, image brightness, image contrast, and image random rotation;
[0030] 103: Use the Weighted-Entropy evaluation standard for active learning, sort the samples to be labeled in increasing order according to the Weighted-Entropy value, select the highest K samples for labeling, and add them to the training set as training samples;
[0031] 104: Use the pseudo-labeling algorithm to sort the samples to be labeled in increasing order according to Entropy (information entropy), select the lowest H samples, and use the prediction results of the model as p...
Embodiment 2
[0035] The scheme in embodiment 1 is further introduced below in conjunction with specific examples, see the following description for details:
[0036] 1. Dataset introduction:
[0037] The data set used in this method is the data of the first round of the Guangdong Industrial Intelligent Manufacturing Big Data Innovation Competition in the Tianchi Intelligent Algorithm Competition (hereinafter referred to as "aluminum data set"). The aluminum data set mainly includes twelve categories, eleven of which are defect categories, and one category is normal (non-defect) category. The defect categories are "non-conductive", "scratch", "horizontal bar indentation", "orange peel", "bottom leak", "bruise", "pit", "convex powder", "coating Cracks”, “Dirty Spots” and “Other Defects” in eleven categories. Among them, "other defects" also include "deformation", "barge", "white spot", "grinding print", "back bottom", "scratch", "crater", "corner leak bottom", "Aluminum chips", "jet flow"...
Embodiment 3
[0068] Below in conjunction with concrete experimental data, the scheme in embodiment 1 and 2 is carried out feasibility verification, see the following description for details:
[0069]First, the embodiment of the present invention uses the ImageNet data set to pre-train the SEResNet-152 network, so that the model has preliminary feature extraction and classification capabilities. Then randomly select 5% of the data in the training set of the aluminum data set after data enhancement and give labeling information to join the labeled data pool L as training data to initialize the model; and the remaining 95% of the training data Then, as samples to be labeled, stored in the data pool U to be labeled.
[0070] After the initialization training is completed, input the data in the data pool U to be labeled into the model one at a time, calculate the Weighted-Entropy score of each sample according to formula (1), and select the highest Weighted-Entropy score of 295 (total 5% of th...
PUM
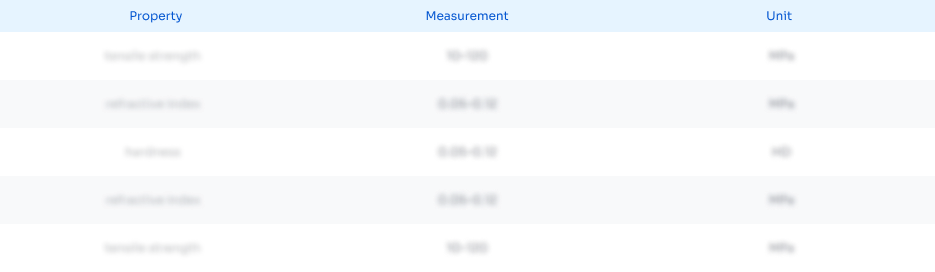
Abstract
Description
Claims
Application Information
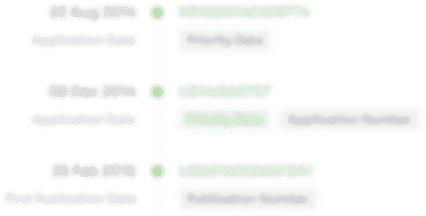
- R&D Engineer
- R&D Manager
- IP Professional
- Industry Leading Data Capabilities
- Powerful AI technology
- Patent DNA Extraction
Browse by: Latest US Patents, China's latest patents, Technical Efficacy Thesaurus, Application Domain, Technology Topic.
© 2024 PatSnap. All rights reserved.Legal|Privacy policy|Modern Slavery Act Transparency Statement|Sitemap