Weld seam and weld seam defect detection method based on deep learning
A defect detection and deep learning technology, which is applied in the direction of optical test defects/defects, can solve the problem that the type and position of weld defects cannot be effectively detected, and achieve the effect of increasing accuracy and classifying accurately
- Summary
- Abstract
- Description
- Claims
- Application Information
AI Technical Summary
Problems solved by technology
Method used
Image
Examples
Embodiment Construction
[0042] The technical solution of the present invention will be described in detail below in conjunction with the drawings and embodiments.
[0043] A method for detecting welds and weld defects based on deep learning, using YOLO V3 network to achieve weld and / or weld defect detection; (YOLO V3 network includes input layer, interconnected convolutional layer, activation function layer, dropout Layer, residual layer, fully connected layer, softmax logic output layer; between two adjacent layers, the output value of the previous level is used as the input value of the next level.)
[0044] The YOLO V3 network for weld and / or weld defect detection is trained through the following steps:
[0045] 1) Use the rectangular positioning frame to select and mark the welds of the workpiece images containing the welds, and use 5000 such images as the training data set;
[0046] As an embodiment of the present invention, a single workpiece image contains 2-15 weld seam areas;
[0047] The weld image ...
PUM
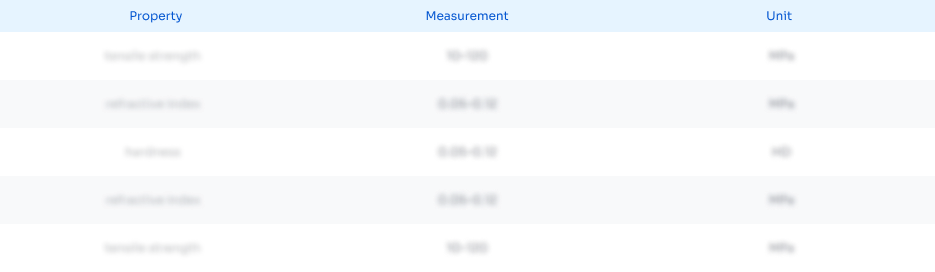
Abstract
Description
Claims
Application Information
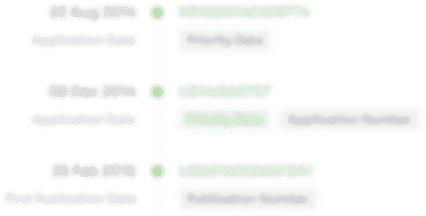
- R&D
- Intellectual Property
- Life Sciences
- Materials
- Tech Scout
- Unparalleled Data Quality
- Higher Quality Content
- 60% Fewer Hallucinations
Browse by: Latest US Patents, China's latest patents, Technical Efficacy Thesaurus, Application Domain, Technology Topic, Popular Technical Reports.
© 2025 PatSnap. All rights reserved.Legal|Privacy policy|Modern Slavery Act Transparency Statement|Sitemap|About US| Contact US: help@patsnap.com