A method for improving a generative adversarial network by using adaptive control learning
An adaptive control and network technology, applied in neural learning methods, biological neural network models, etc., can solve problems that hinder effective learning of generators, unbalanced capabilities of generator and discriminator models, and unbalanced capabilities of generator and discriminator models Growth and other issues, to achieve the effect of improving training stability, expanding the available range, and improving stability
- Summary
- Abstract
- Description
- Claims
- Application Information
AI Technical Summary
Problems solved by technology
Method used
Image
Examples
Embodiment
[0072] 1), against the generative network
[0073] GANs estimate generative models through an adversarial process, where a generator G plays a game against a discriminator D. The input to D comes from two data distributions: real data and synthetic data, the latter being generated by G. D is trained to maximize its ability to fully classify samples as real or synthetic, while G is trained to minimize D's ability to discern synthetic data. In the original framework, the training objective is defined as a maximin problem:
[0074]
[0075] where G is the mapping of the input noise variable Pz(z) to the generated data distribution P g and D is a function that maps the data space to scalar values, where each value represents the probability that a particular sample comes from the actual data distribution. Functions G and D constitute the GAN network, which is usually a neural network model and is trained simultaneously on its objective function. Loss function L for G and D ...
PUM
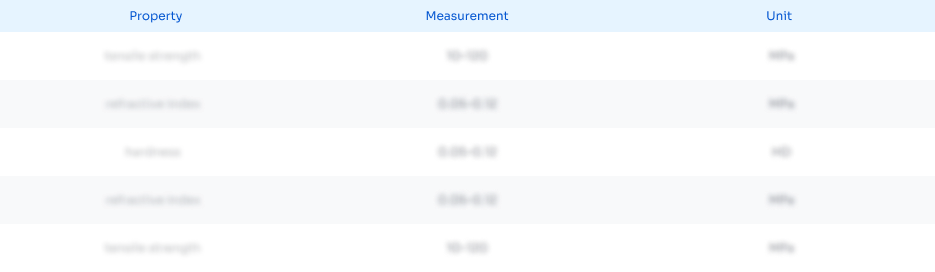
Abstract
Description
Claims
Application Information
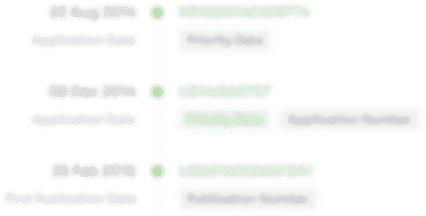
- R&D Engineer
- R&D Manager
- IP Professional
- Industry Leading Data Capabilities
- Powerful AI technology
- Patent DNA Extraction
Browse by: Latest US Patents, China's latest patents, Technical Efficacy Thesaurus, Application Domain, Technology Topic.
© 2024 PatSnap. All rights reserved.Legal|Privacy policy|Modern Slavery Act Transparency Statement|Sitemap