Mechanical equipment health state identification method based on embedded cycle network
A technology for mechanical equipment and health status, applied in character and pattern recognition, neural learning methods, testing of mechanical components, etc., can solve the problems of weak diagnosis ability, weakened mechanical equipment diagnosis ability, lack of information mining, etc. , the effect of weakening the influence
- Summary
- Abstract
- Description
- Claims
- Application Information
AI Technical Summary
Problems solved by technology
Method used
Image
Examples
Embodiment 1
[0025] Taking the health state identification of rolling bearings as an example, the feasibility of the present invention is verified.
[0026] Step 1: Analyze the experimental data of a rolling bearing. The bearing specification used in the experiment is JEM SKF6025-2RS deep groove ball bearing, and the sampling frequency is 48K / s. The faults to be dealt with are artificially processed blind holes of a certain size on the surface of each component. In this invention, the detailed description of the data set used for testing is shown in Table 1. The original dataset was extended by adding white noise with different signal-to-noise ratios. per data set Contains bearing health for 10 different fault types. The number of samples in the three loads 1-3 is the same for each health state, and each sample contains 10,000 data points and is divided into 5 segments to form a new form One of the five samples is used as test data, and the remaining samples are used as training data....
PUM
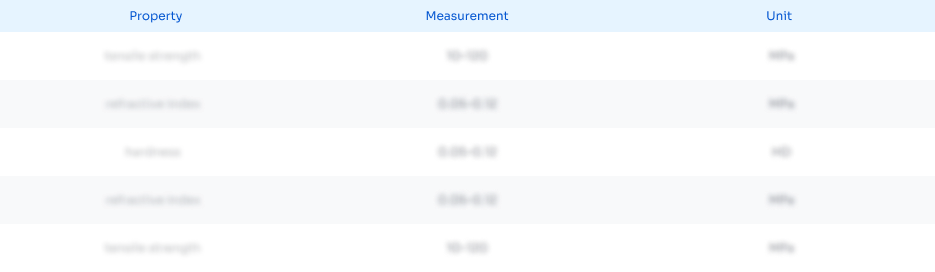
Abstract
Description
Claims
Application Information
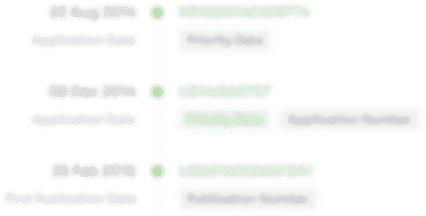
- R&D Engineer
- R&D Manager
- IP Professional
- Industry Leading Data Capabilities
- Powerful AI technology
- Patent DNA Extraction
Browse by: Latest US Patents, China's latest patents, Technical Efficacy Thesaurus, Application Domain, Technology Topic.
© 2024 PatSnap. All rights reserved.Legal|Privacy policy|Modern Slavery Act Transparency Statement|Sitemap