Multi-agent reinforcement learning scheduling method and system, and electronic device
A reinforcement learning and multi-agent technology, applied in the field of multi-agent systems, can solve problems such as strategies without coordination, resource allocation that cannot be realized, and training difficulties
- Summary
- Abstract
- Description
- Claims
- Application Information
AI Technical Summary
Problems solved by technology
Method used
Image
Examples
Embodiment Construction
[0044] In order to make the purpose, technical solution and advantages of the present application clearer, the present application will be further described in detail below in conjunction with the accompanying drawings and embodiments. It should be understood that the specific embodiments described here are only used to explain the present application, not to limit the present application.
[0045] In order to solve the deficiencies in the existing technology, the multi-agent reinforcement learning scheduling method of the embodiment of the application uses the multi-agent reinforcement learning technology in the field of reinforcement learning, according to the load information on each service node in the cloud service environment Modeling, using cyclic neural network to learn timing information to make decisions, training an agent for each server, and competing or cooperating among agents with different tasks to maintain load balancing under the entire network topology. Afte...
PUM
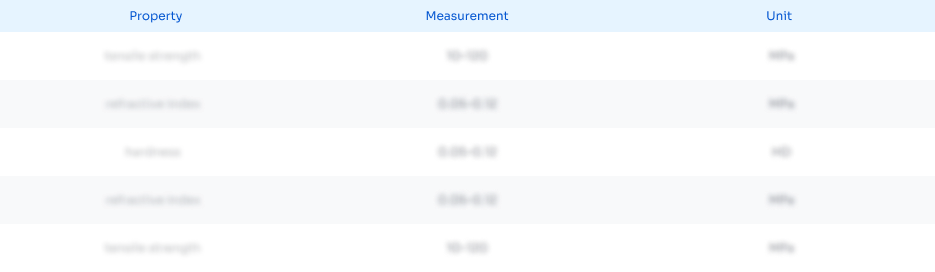
Abstract
Description
Claims
Application Information
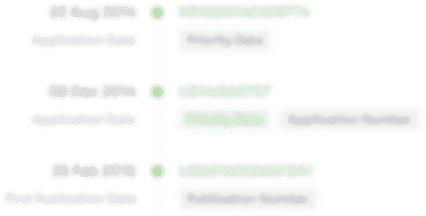
- R&D Engineer
- R&D Manager
- IP Professional
- Industry Leading Data Capabilities
- Powerful AI technology
- Patent DNA Extraction
Browse by: Latest US Patents, China's latest patents, Technical Efficacy Thesaurus, Application Domain, Technology Topic.
© 2024 PatSnap. All rights reserved.Legal|Privacy policy|Modern Slavery Act Transparency Statement|Sitemap