Inspection robot target positioning method based on deep learning framework
An inspection robot and deep learning technology, applied in the direction of neural learning methods, instruments, manipulators, etc., can solve the problem of inability to judge whether the inspection image contains equipment targets, reduce the accuracy of feature point coordinate mapping between images, and the robot cannot recognize the equipment area And other issues
- Summary
- Abstract
- Description
- Claims
- Application Information
AI Technical Summary
Problems solved by technology
Method used
Image
Examples
Embodiment Construction
[0037] The following will clearly and completely describe the technical solutions in the embodiments of the present invention with reference to the accompanying drawings in the embodiments of the present invention. Obviously, the described embodiments are only some, not all, embodiments of the present invention.
[0038] refer to Figure 1-3 , a method for target location of an inspection robot based on a deep learning framework, comprising the following steps:
[0039] S1. Configure the device tree in the robot inspection scene, divide the device type, and perform tree classification for each device. In the instrument device category of the root node, branch nodes such as pointer instruments and digital display instruments can be divided into subcategories. Type instruments can be divided into leaf nodes such as rectangular floating pointers and arc-shaped axis pointers. Each device to be tested has a category.
[0040] S2. Collect and make a sample image of each leaf node t...
PUM
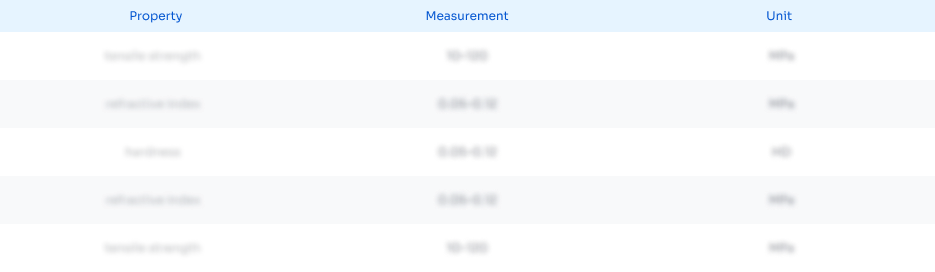
Abstract
Description
Claims
Application Information
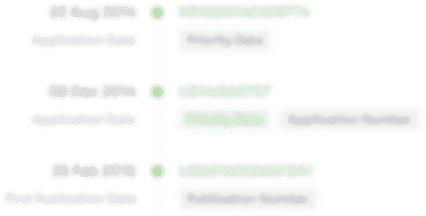
- R&D Engineer
- R&D Manager
- IP Professional
- Industry Leading Data Capabilities
- Powerful AI technology
- Patent DNA Extraction
Browse by: Latest US Patents, China's latest patents, Technical Efficacy Thesaurus, Application Domain, Technology Topic.
© 2024 PatSnap. All rights reserved.Legal|Privacy policy|Modern Slavery Act Transparency Statement|Sitemap