A Noise Removal Method for Seismic Data Based on Perceptron Residual Autoencoder Network
A technology of self-encoding network and seismic data, which is applied in the field of seismic data denoising of perceptron residual self-encoding network, can solve the problems of few seismic denoising methods and cannot suppress multiple waves, and achieves preservation of local details and short training time. , the effect of fast convergence
- Summary
- Abstract
- Description
- Claims
- Application Information
AI Technical Summary
Problems solved by technology
Method used
Image
Examples
Embodiment Construction
[0064] In order to efficiently remove multiple waves and random noise in seismic data, the multi-layer perceptron residual convolution auto-encoding block and auto-encoding network are the basic network structures, and the denoising perceptron residual auto-encoding network model is proposed, such as image 3 Shown. Reference figure 1 , The flowchart of the present invention shown includes the following steps.
[0065] Step 1. Make training set and test set, the specific steps are as follows:
[0066] (1) Process the original seismic data with a size of 200×92 into a common center point gather after dynamic correction;
[0067] (2) In order to demonstrate that the present invention can simultaneously remove random noise and multiple waves, if the original seismic data does not contain random noise or contains less random noise, this example will increase the content of random noise accordingly;
[0068] (3) Make a training set by taking the original data with noise and the seismic da...
PUM
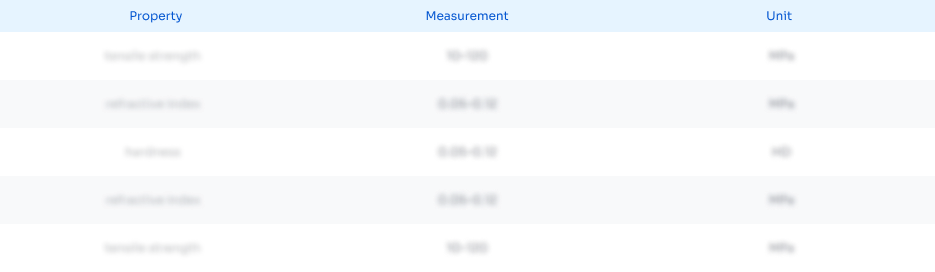
Abstract
Description
Claims
Application Information
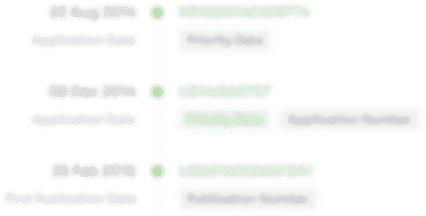
- R&D Engineer
- R&D Manager
- IP Professional
- Industry Leading Data Capabilities
- Powerful AI technology
- Patent DNA Extraction
Browse by: Latest US Patents, China's latest patents, Technical Efficacy Thesaurus, Application Domain, Technology Topic, Popular Technical Reports.
© 2024 PatSnap. All rights reserved.Legal|Privacy policy|Modern Slavery Act Transparency Statement|Sitemap|About US| Contact US: help@patsnap.com