Selective hierarchical integration Gaussian process regression soft measurement modeling method based on evolutionary multi-objective optimization
A Gaussian process regression and multi-objective optimization technology, applied in the field of soft sensing, can solve the problems of not considering the accuracy of the base model and the balance of diversity, and the poor effect of the integrated model, so as to improve the performance of the model, reduce the complexity, Guaranteeing the effect of accuracy and diversity
- Summary
- Abstract
- Description
- Claims
- Application Information
AI Technical Summary
Problems solved by technology
Method used
Image
Examples
Embodiment 1
[0020] Embodiment 1: as figure 1 As shown, in this embodiment, taking the TE (Tennessee Eastman Process) chemical process as an example, 22 continuous measurement variables and 12 manipulated variables are selected as the original input, and the E component concentration in Stream 9 is used as the output of the soft sensor model .
[0021] Step 1: Collect input and output samples and divide them into training set (50%), verification set (25%), and test set (25%).
[0022] Step 2: Obtain a set of diversity modeling sample subsets {(X 1 ,y 1 );…;(X M ,y M )}, and then conduct PMI correlation analysis on each modeling sample subset to construct a set of diversity input subspace {S 1 ,...,S M}, the specific implementation content of the PMI guidelines is:
[0023] For a subset of Bootstrapping modeling samples, the KNN estimation method is used to estimate the PMI value and the K-fold cross-validation and permutation testing methods are used to determine the best nearest ne...
PUM
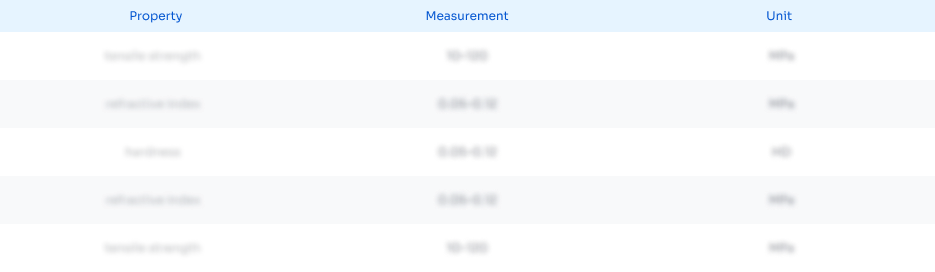
Abstract
Description
Claims
Application Information
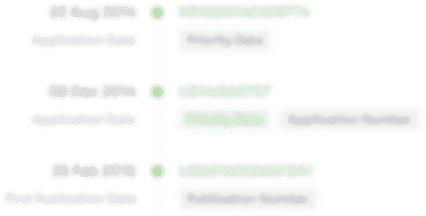
- R&D
- Intellectual Property
- Life Sciences
- Materials
- Tech Scout
- Unparalleled Data Quality
- Higher Quality Content
- 60% Fewer Hallucinations
Browse by: Latest US Patents, China's latest patents, Technical Efficacy Thesaurus, Application Domain, Technology Topic, Popular Technical Reports.
© 2025 PatSnap. All rights reserved.Legal|Privacy policy|Modern Slavery Act Transparency Statement|Sitemap|About US| Contact US: help@patsnap.com