Photovoltaic power prediction method based on a convolutional neural network and meta-learning
A convolutional neural network and power prediction technology, which is applied in the field of renewable energy development and utilization, can solve the problems of few research methods, high cost, and poor accuracy, and achieve the effects of small prediction error index, improved prediction accuracy, and high accuracy
- Summary
- Abstract
- Description
- Claims
- Application Information
AI Technical Summary
Problems solved by technology
Method used
Image
Examples
Embodiment Construction
[0051] The present invention will be further described below in conjunction with the accompanying drawings and specific embodiments.
[0052] Such as figure 1 As shown, the photovoltaic power prediction method based on convolutional neural network and meta-learning, the method specifically includes the following steps:
[0053] Data preprocessing: two-step decomposition of historical photovoltaic power data; dimension conversion of photovoltaic power series; analysis of weather types on the day to be predicted based on radiation data in numerical weather forecast;
[0054] Photovoltaic power point prediction: Establish a deep convolutional neural network model, namely the residual network (ResNet), which takes historical photovoltaic power data, historical meteorological data and numerical weather forecast data as model input, and takes the photovoltaic power of the day to be predicted as output to form a Model training samples; select similar day training samples, based on t...
PUM
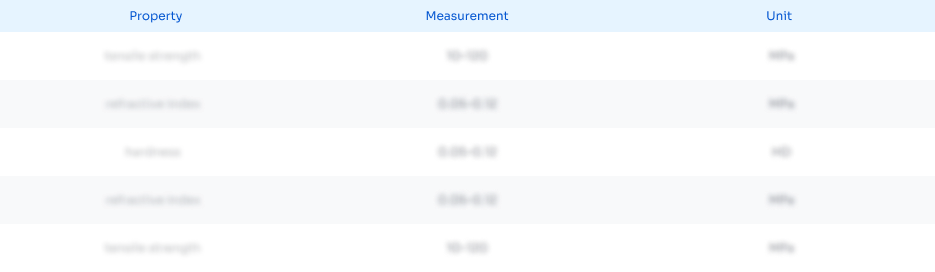
Abstract
Description
Claims
Application Information
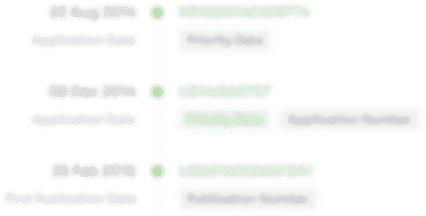
- Generate Ideas
- Intellectual Property
- Life Sciences
- Materials
- Tech Scout
- Unparalleled Data Quality
- Higher Quality Content
- 60% Fewer Hallucinations
Browse by: Latest US Patents, China's latest patents, Technical Efficacy Thesaurus, Application Domain, Technology Topic, Popular Technical Reports.
© 2025 PatSnap. All rights reserved.Legal|Privacy policy|Modern Slavery Act Transparency Statement|Sitemap|About US| Contact US: help@patsnap.com