Image recognition method combining convolutional neural network and gradient lifting tree
A convolutional neural network and gradient boosting tree technology, applied in the field of image analysis, can solve problems such as poor model generalization ability, deep learning model overfitting, and difficulty in adapting to large-scale data training, and achieve the effect of improving classification accuracy.
- Summary
- Abstract
- Description
- Claims
- Application Information
AI Technical Summary
Problems solved by technology
Method used
Image
Examples
Embodiment Construction
[0063] The present invention will be further described below in conjunction with the accompanying drawings and specific embodiments of the present invention. The specific embodiments described here are only for explaining the present invention, rather than limiting the present invention. In addition, for ease of description, the drawings only show some implementations of the present invention, rather than all implementations.
[0064] The present invention will be further described below in conjunction with the accompanying drawings and specific embodiments.
[0065] The present invention is an image recognition method combining a convolutional neural network and a gradient boosting tree. Taking breast cancer image recognition and classification as an example, the input images are divided into normal tissues, benign lesions, and cancerous changes. Such as figure 1 As shown, the acquired CT or MRI scan data is imported into this system, the image patch of the input image is ge...
PUM
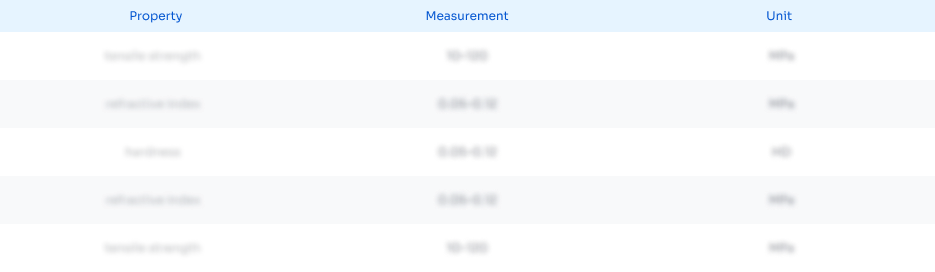
Abstract
Description
Claims
Application Information
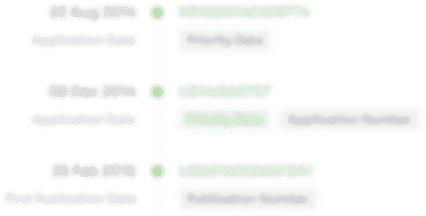
- R&D Engineer
- R&D Manager
- IP Professional
- Industry Leading Data Capabilities
- Powerful AI technology
- Patent DNA Extraction
Browse by: Latest US Patents, China's latest patents, Technical Efficacy Thesaurus, Application Domain, Technology Topic.
© 2024 PatSnap. All rights reserved.Legal|Privacy policy|Modern Slavery Act Transparency Statement|Sitemap