Deep neural network test sufficiency method based on variable intensity combination test
A deep neural network and combined testing technology, applied in the field of deep learning testing, can solve problems such as large amount of data, complex use scenarios, frequent security incidents, etc., to achieve the effect of ensuring adequacy and improving credibility
- Summary
- Abstract
- Description
- Claims
- Application Information
AI Technical Summary
Problems solved by technology
Method used
Image
Examples
Embodiment Construction
[0029] Below in conjunction with accompanying drawing and specific embodiment, further illustrate the present invention, should be understood that these examples are only for illustrating the present invention and are not intended to limit the scope of the present invention, after having read the present invention, those skilled in the art will understand various aspects of the present invention All modifications of the valence form fall within the scope defined by the appended claims of the present application.
[0030] A deep neural network test adequacy method based on variable strength combination testing, using variable strength combination testing technology, extracting the relationship between neurons in the deep neural network according to the model weight, extracting neuron combinations with different strengths, according to the neural network The neuron activation state in the unit combination, evaluate the neuron activation state coverage in the neural network, such ...
PUM
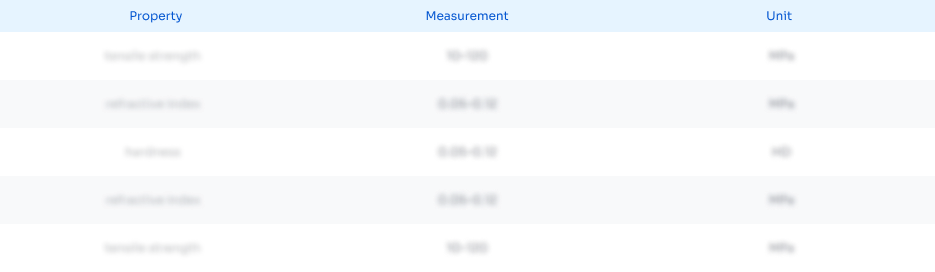
Abstract
Description
Claims
Application Information
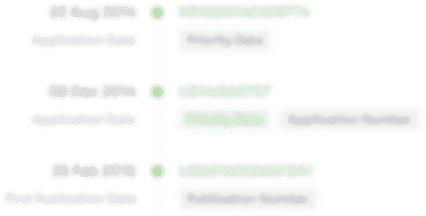
- R&D Engineer
- R&D Manager
- IP Professional
- Industry Leading Data Capabilities
- Powerful AI technology
- Patent DNA Extraction
Browse by: Latest US Patents, China's latest patents, Technical Efficacy Thesaurus, Application Domain, Technology Topic, Popular Technical Reports.
© 2024 PatSnap. All rights reserved.Legal|Privacy policy|Modern Slavery Act Transparency Statement|Sitemap|About US| Contact US: help@patsnap.com