Adaptive threshold channel occupation state prediction method based on LSTM neural network
An adaptive threshold and channel occupancy technology, applied in neural learning methods, biological neural network models, network planning, etc., can solve problems such as inability to capture nonlinear relationships, great influence on model performance, and feature selection dependence, etc. Redundancy, improving spectrum resource utilization, avoiding the effect of noise uncertainty interference
- Summary
- Abstract
- Description
- Claims
- Application Information
AI Technical Summary
Problems solved by technology
Method used
Image
Examples
Embodiment Construction
[0045] The present invention will be further described below in conjunction with the accompanying drawings.
[0046] Such as figure 1 Shown is an adaptive threshold channel occupancy state prediction method based on LSTM neural network, including five parts: determining the adaptive quantization threshold, determining the length of the historical sequence, generating model input and output sets, optimizing model hyperparameters, and real-time spectrum prediction. as follows.
[0047] 1. Determine the adaptive quantization threshold
[0048] By estimating the probability density of the power spectral density time series of each channel in the recent historical spectrum data, the quantization threshold is set for the self-adaptation of different channels.
[0049] Such as figure 2 As shown, for the power spectral density time series x of the i-th channel i Perform kernel density estimation to get x i The probability density estimate for
[0050]
[0051] Among them: ...
PUM
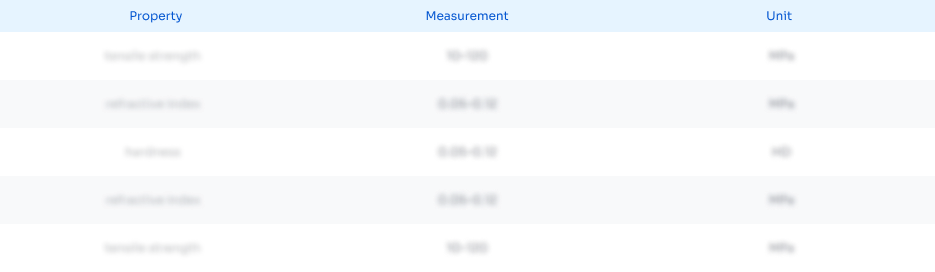
Abstract
Description
Claims
Application Information
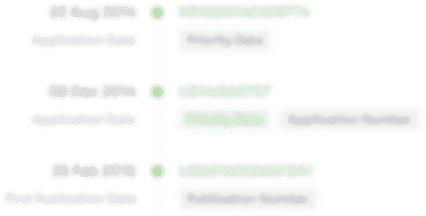
- R&D
- Intellectual Property
- Life Sciences
- Materials
- Tech Scout
- Unparalleled Data Quality
- Higher Quality Content
- 60% Fewer Hallucinations
Browse by: Latest US Patents, China's latest patents, Technical Efficacy Thesaurus, Application Domain, Technology Topic, Popular Technical Reports.
© 2025 PatSnap. All rights reserved.Legal|Privacy policy|Modern Slavery Act Transparency Statement|Sitemap|About US| Contact US: help@patsnap.com