Improved sparse Bayesian learning ISAR imaging scattering coefficient estimation method
A technique of sparse Bayesian and scattering coefficients, which is applied in the field of improved sparse Bayesian learning inverse synthetic aperture radar imaging scattering coefficient estimation, can solve problems such as unsatisfactory requirements, and achieve reduced computational complexity, clear imaging, and reduced matrix effect of scale
- Summary
- Abstract
- Description
- Claims
- Application Information
AI Technical Summary
Problems solved by technology
Method used
Image
Examples
Embodiment 1
[0128] Embodiment 1: This example verifies the effectiveness of the improved sparse Bayesian learning ISAR imaging method proposed by the present invention. The method proposed by the present invention is compared with the range Doppler method, the traditional sparse Bayesian learning method and the matching pursuit method, and all methods perform imaging under the same conditions. The experiment uses the electromagnetic scattering simulation data of a certain type of fighter. The center frequency is 10GHz, and the transmission signal bandwidth is 0.5GHz; the pitch angle is 30°, and the azimuth angle is 0°; Take 64 points for each corner. Imaging results compared to figure 2 shown.
Embodiment 2
[0129] Embodiment 2: This example verifies the effectiveness of the improved sparse Bayesian learning ISAR imaging method proposed by the present invention. The method proposed by the present invention is compared with the range Doppler method, the traditional sparse Bayesian learning method and the matching pursuit method, and all methods perform imaging under the same conditions. The experiment uses the electromagnetic scattering simulation data of a certain type of fighter. The center frequency is 10GHz, and the transmission signal bandwidth is 0.5GHz; the pitch angle is 45°, and the azimuth angle is 45°; Take 64 points for each corner. Imaging results compared to image 3 shown.
[0130] From the comparison of the experimental results in the two embodiments, it can be seen that compared with the range Doppler method, the strong scattering points of the present invention are more obvious, and the image is clearer. Compared with the matching pursuit method and the traditi...
PUM
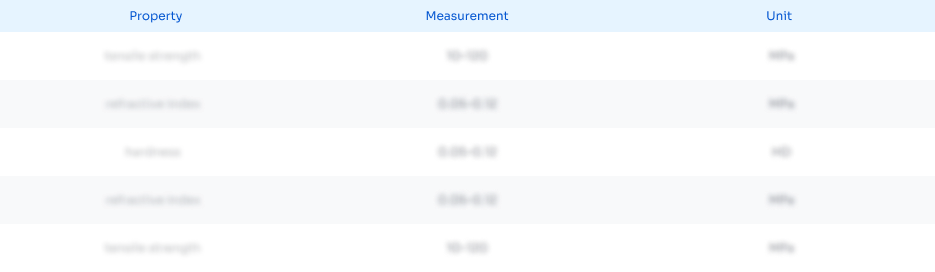
Abstract
Description
Claims
Application Information
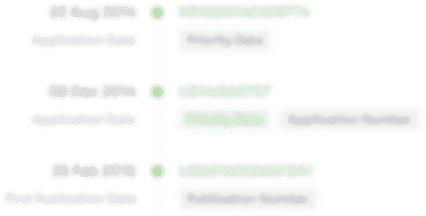
- R&D Engineer
- R&D Manager
- IP Professional
- Industry Leading Data Capabilities
- Powerful AI technology
- Patent DNA Extraction
Browse by: Latest US Patents, China's latest patents, Technical Efficacy Thesaurus, Application Domain, Technology Topic, Popular Technical Reports.
© 2024 PatSnap. All rights reserved.Legal|Privacy policy|Modern Slavery Act Transparency Statement|Sitemap|About US| Contact US: help@patsnap.com