Image semantic segmentation method of guiding feature fusion based on attention mechanism
A technology of feature fusion and semantic segmentation, which is applied to computer components, instruments, biological neural network models, etc., can solve problems such as blurred boundaries and outlines, and achieve the effect of reducing ambiguity, high accuracy, and clear boundary outlines
- Summary
- Abstract
- Description
- Claims
- Application Information
AI Technical Summary
Problems solved by technology
Method used
Image
Examples
Embodiment Construction
[0023] Such as figure 1 As shown, the present invention is based on the attention mechanism to guide the image semantic segmentation method of feature fusion, comprising the following steps:
[0024] (10) Encoder basic network construction: use the improved ResNet-101 to generate a series of features ranging from high resolution and low semantics to low resolution and high semantics;
[0025] Such as figure 2 As shown, the (10) encoder basic network construction steps include:
[0026] (11) Re-deployment of the number of layers of building blocks: redeploy the number of building blocks owned by each stage from res-2 to res-5, and res-2 to res-5 of the original ResNet-101 {3, 4, 23, 3 } The number of building blocks is adjusted to {8, 8, 9, 8};
[0027] The purpose of the convolutional network encoder is to generate a series of features ranging from high-resolution low-semantic to low-resolution high-semantic. The base network usually uses existing convolutional neural netw...
PUM
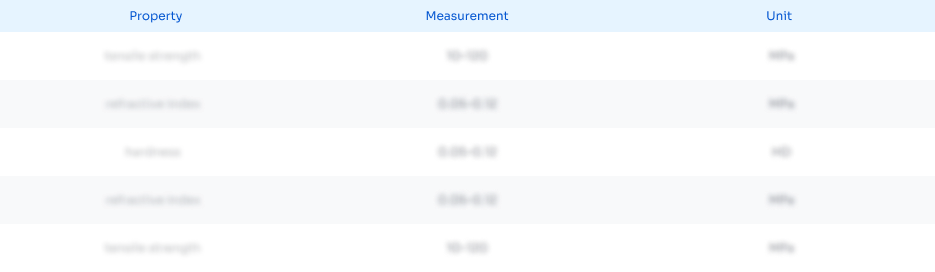
Abstract
Description
Claims
Application Information
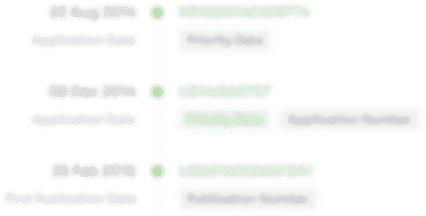
- R&D Engineer
- R&D Manager
- IP Professional
- Industry Leading Data Capabilities
- Powerful AI technology
- Patent DNA Extraction
Browse by: Latest US Patents, China's latest patents, Technical Efficacy Thesaurus, Application Domain, Technology Topic, Popular Technical Reports.
© 2024 PatSnap. All rights reserved.Legal|Privacy policy|Modern Slavery Act Transparency Statement|Sitemap|About US| Contact US: help@patsnap.com