XGBoost soft measurement modeling method based on parallel LSTM auto-encoder dynamic feature extraction
A technology of dynamic features and modeling methods, applied in neural learning methods, instruments, character and pattern recognition, etc., can solve the problems of difficult process dynamics, process nonlinearity, high data dimensions, etc., to speed up training and improve robustness. Sticky, easy-to-migrate effects
- Summary
- Abstract
- Description
- Claims
- Application Information
AI Technical Summary
Problems solved by technology
Method used
Image
Examples
Embodiment
[0082] The beneficial effects brought by the present invention will be described below in conjunction with a specific example of a debutanizer. The debutanizer process is a commonly used standard industrial process platform for the verification of soft-sensing modeling algorithms, and it is the process of removing propane and butane from naphtha gas in industrial refinery processes. The bottom butane content requires soft-sensing techniques for estimation. Image 6 The structure of the debutanizer process is described in . Table 1 shows that the seven auxiliary variables selected for the key quality variable butane content are column top temperature, column top pressure, reflux flow rate, next stage flow rate, temperature of sensitive plate, column bottom temperature and column bottom pressure. For this process, 2345 data were collected continuously at equal time intervals, of which 1563 data were used as training sample sets for modeling, and the other 782 data samples were ...
PUM
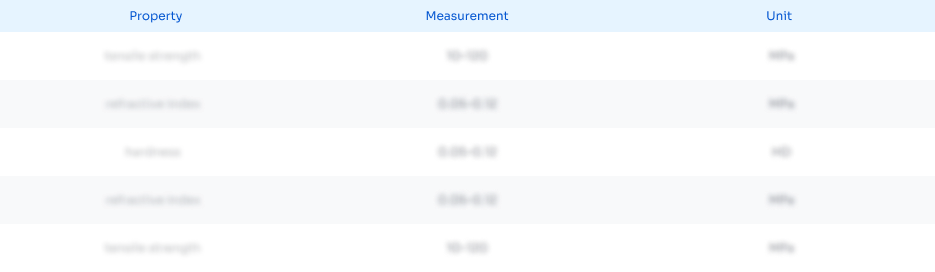
Abstract
Description
Claims
Application Information
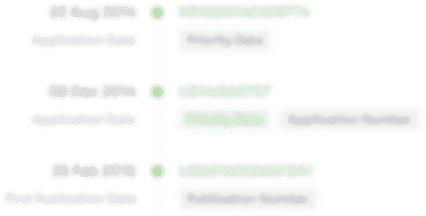
- R&D
- Intellectual Property
- Life Sciences
- Materials
- Tech Scout
- Unparalleled Data Quality
- Higher Quality Content
- 60% Fewer Hallucinations
Browse by: Latest US Patents, China's latest patents, Technical Efficacy Thesaurus, Application Domain, Technology Topic, Popular Technical Reports.
© 2025 PatSnap. All rights reserved.Legal|Privacy policy|Modern Slavery Act Transparency Statement|Sitemap|About US| Contact US: help@patsnap.com