Flight passenger flow rate prediction method based on multi-granularity time attention mechanism
A technology of attention and passenger load factor, applied in forecasting, neural learning methods, instruments, etc., can solve problems that cannot be considered at the same time
- Summary
- Abstract
- Description
- Claims
- Application Information
AI Technical Summary
Problems solved by technology
Method used
Image
Examples
Embodiment 1
[0050] The embodiment of the present invention proposes a recurrent neural network model (MTA-RNN) based on a multi-granularity temporal attention mechanism, figure 1 An architecture diagram of the above-mentioned MTA-RNN model provided for the embodiment of the present invention, the model constructs a multi-level attention mechanism to obtain the time series correlation of the flight passenger load factor at different time granularities, the model includes: combined with the attention mechanism at the time of departure The encoder and the decoder combined with the off-day attention mechanism.
[0051] The encoder that combines the attention mechanism at the moment of take-off includes a hierarchical structure of the first layer of long short-term memory network LSTM unit and the second layer of LSTM unit, the first layer of LSTM unit is figure 1 The LSTM unit below the encoder in , and the second layer LSTM unit is figure 1 LSTM unit above the encoder in . The historical p...
Embodiment 2
[0092] figure 2 This is a comparison chart of economy class occupancy factors of flights with different departure times on the Beijing-Shanghai route in March 2010. The horizontal axis is the flight departure date, and the vertical axis is the economy class occupancy factors of flights. Different curves represent changes in economy class occupancy factors of flights at different departure times curve. From figure 2 It can be seen that the passenger load factor of the flight has the following characteristics:
[0093] 1. Relevance of take-off time. Given a certain route, the passenger load factors of flights with different departure times on a certain departure day affect each other, and the passenger load factors of flights with closer departure times are closer. Depend on figure 2 It can be seen that the daily economy class passenger load factor and the overall passenger load factor curve of the 8:30AM flight and the 9:00AM flight with similar departure times are close...
Embodiment approach
[0098] Taking the real historical flight passenger load factor data set provided by TravelSky as an example, the flight data of the Beijing-Shanghai route with more than 584 (730*89%) departure days within two years is selected as the experimental data set. If the time is set The window length D=28, and the number of forecast days τ=7, then the statistical information is shown in Table 1.
[0099] Table 1 Dataset Statistics
[0100]
[0101] image 3 A processing flowchart of a flight load factor prediction method based on multi-granularity time attention provided by an embodiment of the present invention includes the following processing steps:
[0102] Step S1, constructing experimental samples based on the experimental data set, and dividing the training set, verification set and test set;
[0103]The present invention divides the data set into non-overlapping training, verification and test sets in chronological order, and the division ratio is 8:1:1, that is, 27 flig...
PUM
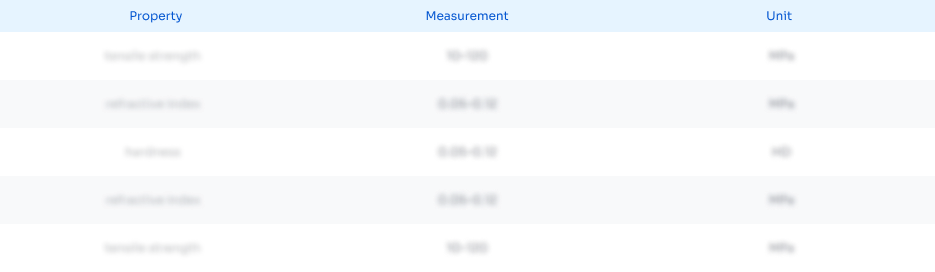
Abstract
Description
Claims
Application Information
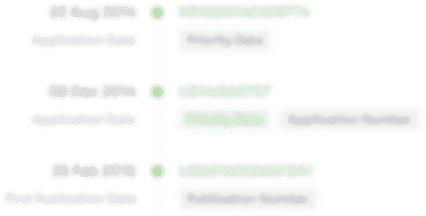
- R&D Engineer
- R&D Manager
- IP Professional
- Industry Leading Data Capabilities
- Powerful AI technology
- Patent DNA Extraction
Browse by: Latest US Patents, China's latest patents, Technical Efficacy Thesaurus, Application Domain, Technology Topic, Popular Technical Reports.
© 2024 PatSnap. All rights reserved.Legal|Privacy policy|Modern Slavery Act Transparency Statement|Sitemap|About US| Contact US: help@patsnap.com