Time sequence Bayesian compression sampling and signal decompression reconstruction method and data loss recovery method
A technology of Bayesian compression and compressed sampling, which is applied in the direction of electrical digital data processing, redundant data error detection in calculation, complex mathematical operations, etc. Issues such as not being fully explored and difficult to maintain stability
- Summary
- Abstract
- Description
- Claims
- Application Information
AI Technical Summary
Problems solved by technology
Method used
Image
Examples
Embodiment Construction
[0090] The technical solutions in the embodiments of the present invention will be clearly and completely described below in conjunction with the accompanying drawings in the embodiments of the present invention. Obviously, the described embodiments are only some of the embodiments of the present invention, not all of them. Based on the embodiments of the present invention, all other embodiments obtained by persons of ordinary skill in the art without making creative efforts belong to the protection scope of the present invention.
[0091] The purpose of the present invention is to solve the problem that the signal decompression and reconstruction of the existing Bayesian compression sampling method is not robust enough at a higher compression rate, which leads to the inability to achieve stable lossless decompression, and proposes a time series Bayesian Compressed sampling and signal decompression reconstruction method. Compressive sampling for 1D time series signals and 2D i...
PUM
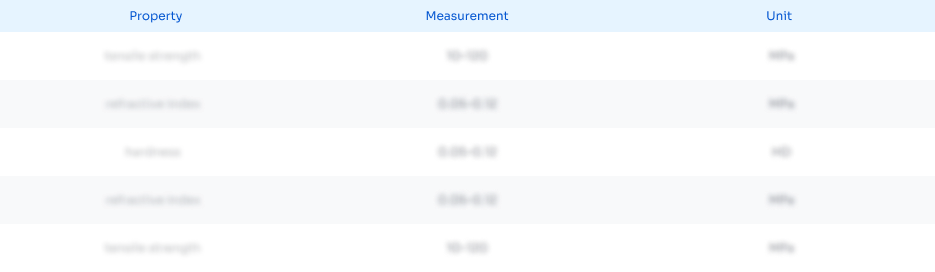
Abstract
Description
Claims
Application Information
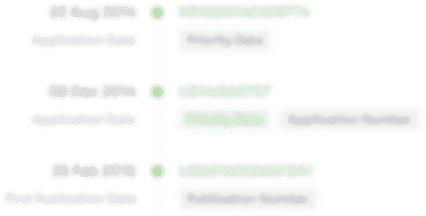
- R&D Engineer
- R&D Manager
- IP Professional
- Industry Leading Data Capabilities
- Powerful AI technology
- Patent DNA Extraction
Browse by: Latest US Patents, China's latest patents, Technical Efficacy Thesaurus, Application Domain, Technology Topic, Popular Technical Reports.
© 2024 PatSnap. All rights reserved.Legal|Privacy policy|Modern Slavery Act Transparency Statement|Sitemap|About US| Contact US: help@patsnap.com