Multi-robot path planning method based on priori knowledge and DQN algorithm
A priori knowledge and multi-robot technology, applied in the direction of instrumentation, calculation, two-dimensional position/channel control, etc., can solve the problems of large training randomness and slow convergence speed of targetQ network
- Summary
- Abstract
- Description
- Claims
- Application Information
AI Technical Summary
Problems solved by technology
Method used
Image
Examples
Embodiment 1
[0098] like figure 1 Shown: A multi-robot path planning method based on prior knowledge and DQN algorithm, including:
[0099] S1: Initialize the multi-robot system iteration number threshold N, exploration step threshold M, time step threshold C, prior knowledge, prior rules, experience pool D, iteration number i, exploration step number t, Q network, and randomly generate Q The label weight ω of the network, the prior knowledge is generated according to the optimal path of each single robot, and the prior rules include the state sequence action sequence special state sequence and prior Q-value vector Q p ; Initialize the Q table and targetQ network through prior knowledge, so that the network weight of the targetQ network
[0100] Specifically, in this embodiment, the state sequence of the preset multi-robot system is The sequence of actions is The special state sequence is When a special state p occurs i In the case of , the optimal action selection strategy...
Embodiment 2
[0111] Embodiment 2: This embodiment also discloses a planning method for an optimal path of a single robot.
[0112] like image 3 Shown: the planning method of the optimal path of a single robot, including:
[0113]S101: Initialize the action set A, state set S, maximum number of iterations n, maximum number of exploration steps m, minimum number of paths MinPathNum, maximum number of successful pathfinding MaxSuccessNum, exploration factor ε, and single update step size eSize of the single-robot system , Exploration factor change cycle eCycle, maximum counting threshold h, start update time B(s, a), complete update time, action value function Q(s, a), state-action visit times C(s, a), reward The function stores U(s, a), the number of times of successful pathfinding SuccessNum, the number of successful paths PathNum, the PathList of successful paths, the storage table List of successful paths, the number of iterations i and the number of exploration steps t.
[0114] S102:...
Embodiment 3
[0139] In this embodiment, a simulation experiment of path planning of a multi-robot system is disclosed.
[0140] 1. Description of the simulation experiment
[0141]1) During the simulation experiment, the software platform uses Windows 10 operating system, the CPU uses Inter Core I5-8400, and the size of the running memory is 16GB. The path planning algorithm of the single robot system will use Python language and TensorFlow deep learning tool to complete the simulation experiment, and the multi-robot path planning algorithm will be written on the matlab2016a simulation software using matlab language.
[0142] 2) This paper will use the grid method to describe the environment, divide the working space of the robot system into several small grids, and each small grid can represent a state of the robot system. In the map, the white grid indicates the safe area, and the black grid indicates the existence of obstacles.
[0143] The target state and obstacles in the environmen...
PUM
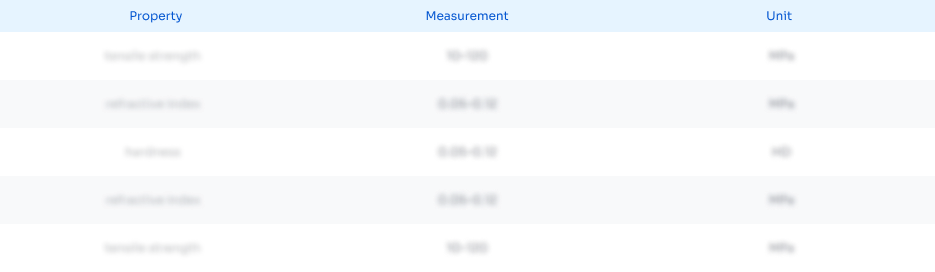
Abstract
Description
Claims
Application Information
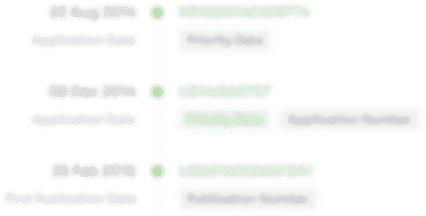
- R&D Engineer
- R&D Manager
- IP Professional
- Industry Leading Data Capabilities
- Powerful AI technology
- Patent DNA Extraction
Browse by: Latest US Patents, China's latest patents, Technical Efficacy Thesaurus, Application Domain, Technology Topic.
© 2024 PatSnap. All rights reserved.Legal|Privacy policy|Modern Slavery Act Transparency Statement|Sitemap