Power transmission line defect detection method based on hierarchical region feature fusion learning
A technology of regional characteristics and transmission lines, which is applied in the field of image analysis, can solve problems such as complex and diverse defects and target background differences, and achieve the effects of strengthening overall perception capabilities, improving efficiency, and saving time for adjusting parameters
- Summary
- Abstract
- Description
- Claims
- Application Information
AI Technical Summary
Problems solved by technology
Method used
Image
Examples
Embodiment 1
[0075] Transmission line defect detection method based on hierarchical regional feature fusion learning, please refer to the attached figure 1 As shown, including: constructing and calling the Faster R-CNN model; regressing the target features extracted by the backbone network through the RPN network to obtain the target area; performing RoI pooling operations on the input image to generate local deep and shallow regional features, and generating them through the depth selection network The weight required for feature fusion fuses the deep feature area and the shallow feature area; and generates the final prediction result through the classification network and the regression network.
[0076] The specific steps are as follows:
[0077] S1. ImageNet-based network pre-training:
[0078] Use the vgg16 network as the deep feature extraction network, pre-train on the ImageNet data set, and use the weight value obtained from the pre-training as the initial parameter value of the m...
Embodiment 2
[0124] The inspection line image is used as the input of the depth detection model, and the pre-trained vgg16 network extracts features, and the depth model generates anchors of different sizes (the size of the anchors is set to (4×4), (8×8), (16 ×16), (32×32), the aspect ratios are 0.5, 1, 2), the region of interest is calculated through the RPN network prediction offset, and the deep image features generated by the vgg16 network are matched with the image shallow features of the feature channel. The layer features are respectively sent to the RoI Pooling layer to obtain deep feature ROIs and shallow feature ROIs. The weight of feature fusion is obtained by the depth selection network, and the fused feature map is generated by the method of weighted sum, and the feature map is visualized to obtain the region-level fusion feature map, such as figure 2 shown.
Embodiment 3
[0126] In this embodiment, on the basis of Embodiment 1 and Embodiment 2, the fusion features obtained in Embodiment 2 are used as the input of the classification network and the regression network respectively, and the category score and bounding box offset are predicted, and the cross-entropy loss function and Smooth L 1 The loss function calculates the loss value and learns through backpropagation. At this time, the batchsize is set to 128, the learning rate decay is set to 0.001, the number of iterations is 40,000, and the candidate frame is selected using the non-maximum suppression method. After the training is completed, input the inspection image to be detected into the depth model, and get image 3 Detected images labeled with predicted classes and predicted bounding boxes in .
[0127] Based on the Faster R-CNN model, the present invention returns the target features extracted by the backbone network through the RPN network to obtain the target area, and performs Ro...
PUM
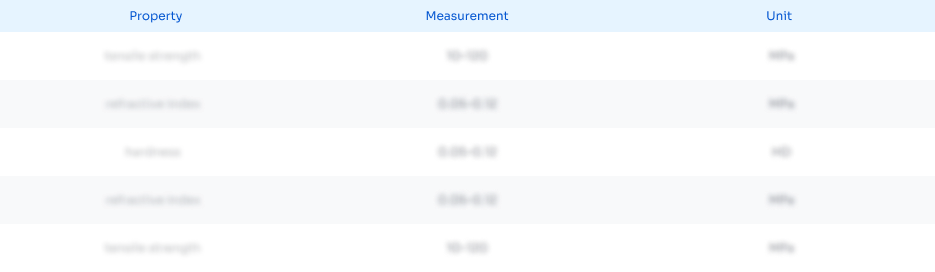
Abstract
Description
Claims
Application Information
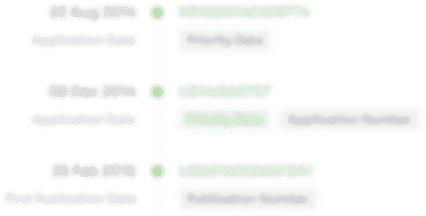
- R&D Engineer
- R&D Manager
- IP Professional
- Industry Leading Data Capabilities
- Powerful AI technology
- Patent DNA Extraction
Browse by: Latest US Patents, China's latest patents, Technical Efficacy Thesaurus, Application Domain, Technology Topic.
© 2024 PatSnap. All rights reserved.Legal|Privacy policy|Modern Slavery Act Transparency Statement|Sitemap