Fault detection method and system based on global preserving unsupervised kernel extreme learning machine
A technology of nuclear extreme learning machine and extreme learning machine, which is applied in the field of fault detection method and system based on global preservation unsupervised nuclear extreme learning machine, can solve the problems affecting the effect of fault detection and achieve the effect of improving the effect
- Summary
- Abstract
- Description
- Claims
- Application Information
AI Technical Summary
Problems solved by technology
Method used
Image
Examples
Embodiment 2
[0170] This embodiment provides a fault detection system based on a globally preserved unsupervised kernel extreme learning machine, including:
[0171] Offline modeling modules, which include:
[0172] A mathematical model building module, which is used to utilize a normalized training data set to construct a global mathematical model that maintains an unsupervised extreme learning machine; the training data in the training data set are all normal operating condition data of a non-linear working process;
[0173] Output weighted matrix solving module, which is used to convert the optimization problem of global preservation unsupervised extreme learning machine into generalized eigenvalue decomposition problem, obtain the mathematical formula expression of generalized eigenvalue decomposition problem, and use the kernel function to update the generalized eigenvalue decomposition problem mathematical formula Representation, calculate the final solution of the weight matrix outp...
Embodiment 3
[0182] This embodiment provides a computer-readable storage medium.
[0183] A computer-readable storage medium in this embodiment, on which a computer program is stored, and when the program is executed by a processor, the figure 1 The steps in the global-preserving unsupervised kernel extreme learning machine-based fault detection method are shown.
Embodiment 4
[0185] This embodiment provides a computer device.
[0186] A computer device in this embodiment includes a memory, a processor, and a computer program stored in the memory and operable on the processor. When the processor executes the program, it realizes figure 1 The steps in the global-preserving unsupervised kernel extreme learning machine-based fault detection method are shown.
[0187] Those skilled in the art should understand that the embodiments of the present disclosure may be provided as methods, systems, or computer program products. Accordingly, the present disclosure may take the form of a hardware embodiment, a software embodiment, or an embodiment combining software and hardware aspects. Furthermore, the present disclosure may take the form of a computer program product embodied on one or more computer-usable storage media (including but not limited to disk storage, optical storage, etc.) having computer-usable program code embodied therein.
PUM
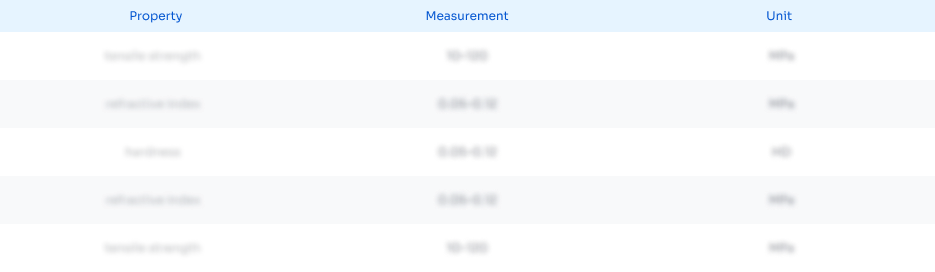
Abstract
Description
Claims
Application Information
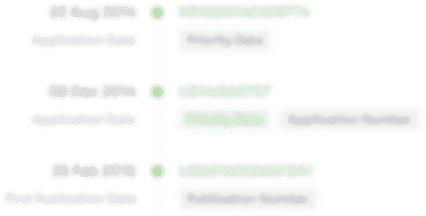
- R&D
- Intellectual Property
- Life Sciences
- Materials
- Tech Scout
- Unparalleled Data Quality
- Higher Quality Content
- 60% Fewer Hallucinations
Browse by: Latest US Patents, China's latest patents, Technical Efficacy Thesaurus, Application Domain, Technology Topic, Popular Technical Reports.
© 2025 PatSnap. All rights reserved.Legal|Privacy policy|Modern Slavery Act Transparency Statement|Sitemap|About US| Contact US: help@patsnap.com