GPU cluster deep learning task parallelization method, device and electronic equipment
A GPU cluster and deep learning technology, applied in the Internet field, can solve problems such as not being able to make full use of GPU resources, not considering the physical characteristics of resources and the characteristics of the task itself, and affecting the resource utilization of GPU clusters, etc.
- Summary
- Abstract
- Description
- Claims
- Application Information
AI Technical Summary
Problems solved by technology
Method used
Image
Examples
Embodiment Construction
[0070] The following will clearly and completely describe the technical solutions in the embodiments of the application with reference to the drawings in the embodiments of the application. Apparently, the described embodiments are only some of the embodiments of the application, not all of them. Based on the embodiments in this application, all other embodiments obtained by persons of ordinary skill in the art without making creative efforts belong to the scope of protection of this application.
[0071] The embodiment of the present application discloses a GPU cluster deep learning task parallelization method, device, electronic equipment, storage medium, and computer program product including instructions, which will be described respectively below.
[0072] The embodiment of this application provides a GPU cluster deep learning task parallelization method, see figure 1 , figure 1 It is a schematic diagram of the GPU cluster deep learning task parallelization method of the...
PUM
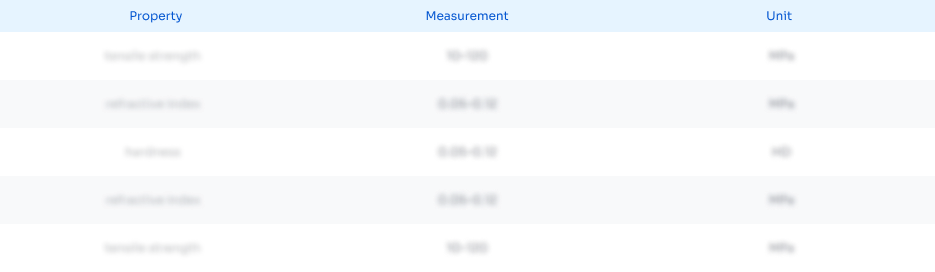
Abstract
Description
Claims
Application Information
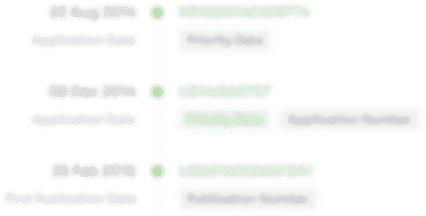
- R&D
- Intellectual Property
- Life Sciences
- Materials
- Tech Scout
- Unparalleled Data Quality
- Higher Quality Content
- 60% Fewer Hallucinations
Browse by: Latest US Patents, China's latest patents, Technical Efficacy Thesaurus, Application Domain, Technology Topic, Popular Technical Reports.
© 2025 PatSnap. All rights reserved.Legal|Privacy policy|Modern Slavery Act Transparency Statement|Sitemap|About US| Contact US: help@patsnap.com